RO1 GM073822 Principal Investigator
Program Director: Borevitz, Justin
GENOME WIDE ASSOCIATION MAPPING IN ARABIDOPSIS THALIANA
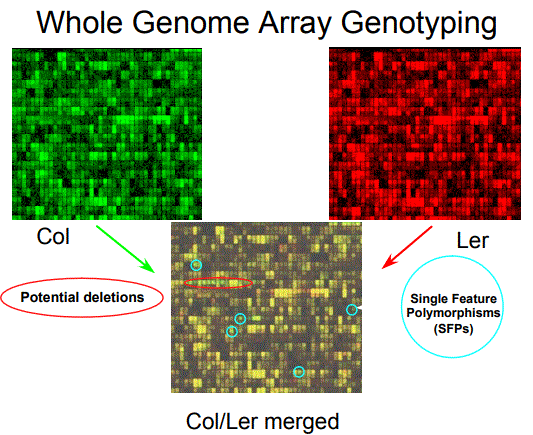
Project Description
Our proposal will investigate the genetic and molecular basis of complex traits and their interactions
with the environment using the model plant Arabidopsis thaliana. We will implement a multi use, high
density oligo-nucleotide tiling array for whole genome resequencing. The sample will include a largely
unstructured core set of 384 wild A. thaliana genomes. This will be used to develop a very high
resolution haplotype map, reveal genome wide patterns of variation, and suggest sites under
natural selection. The ecologically relevant quantitative trait of flowering time will be measured
across two seasonal and two geographic environments which span the native range of A. thaliana.
This and future community phenotypic data will be used to develop and test methods for fine scale
quantitative trait locus (QTL) association scanning capitalizing on the high density haplotype
map. Whole genome association mapping will be developed using coalescent models for detection
and fine mapping. We will determine the functional molecular changes underlying at least one
QTL utilizing the full power of Arabidopsis genetics. Importantly this proposal will develop new
technological inroads for using tiling arrays to generate high density haplotype maps as the
foundation for whole genome association studies. These methods, once established, can then be
extended to other model systems. The development of fine scale linkage disequilibrium mapping
methods will be broadly applicable.
There is a tremendous interest in complex disease association mapping, but much debate over
different approaches and little success to date. The studies proposed here in Arabidopsis will
suggest successful paths for this daunting undertaking, as associations can be quickly confirmed to
identify novel QTL.
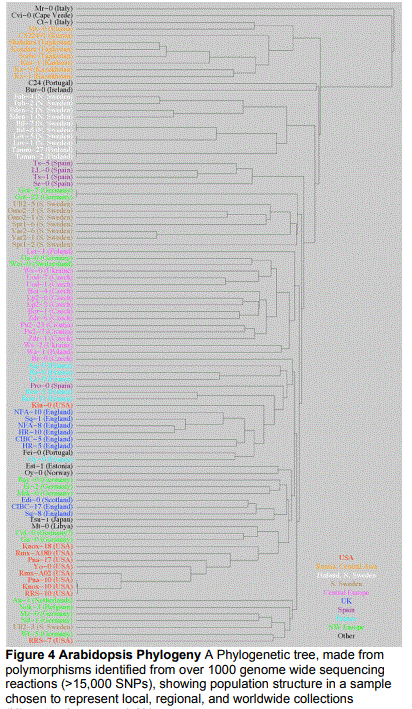
A. Specific Aims
In this proposal we seek to unravel the genetic basis of complex traits by developing methods for full
genome association mapping in the model plant Arabidopsis thaliana. The traits, seedling elongation
and flowering time, are especially sensitive to the environment and are likely to be under natural
selection. We will take advantage of our early access to whole genome oligonucleotide tiling arrays
for comprehensive studies of genetic variation. Single Feature Polymorphism (SFP) resequencing
will be used to develop a complete haplotype map that will be the basis for genome wide association
analysis of quantitative variation. Loci can be finely scanned for rare patterns of variation in
comparison to an empirically defined genome wide distribution. These loci can then be followed up in
further studies and may suggest candidate genes for Quantitative Trait Loci (QTL). We will collect
accurate quantitative phenotypic data from worldwide accessions that span the native geographic
range under seasonal environments recreated in the growth chamber. This and other community
quantitative trait data will be used as a test bed for new methods of linkage disequilibrium mapping
that take advantage of the high density array based haplotype map. QTL identified with association
scans based on coalescent models will be pursued to reveal the molecular genetic basis relying on
the extensive functional genomic resources available in Arabidopsis.
Aim1: Generation of high density haplotype map from 384 world wide accessions.
We will use an oligonucleotide tiling array that covers 72% of the base pairs of the Arabidopsis
genome. This single array (AtTILE1) contains 6.3 million different 25bp oligonucleotides and can be
used for polymorphism or small deletion detection as well as for gene expression including new gene
discovery and alternative splicing (BOREVITZ and ECKER 2004).
A) SFP resequencing. We will select a core set of 384 generally unstructured A. thaliana
accessions for SFP resequencing. A multi stage sampling approach will be used to first survey all
available community accessions at the stock center with 20 SNP markers to identify siblings. A
nonredundant set will be typed with a panel of 384 SNPs to reveal deeper population structure.
The core will be chosen from this data to be made up of equidistantly related individuals with deep
ancestry, representing one or a few regional types. The genotyping will be performed sequentially
with more replication in early stages to confidently identity common SFPs and haplotypes, and
less replication at later stages to genotype SFPs and call rare haplotypes.
B) Evolution, diversity, and selection. The resequencing data should reveal >50% of the intraspecies variation (array coverage is ~72% and sensitivity is >70%). Scans for population genetic
statistics could reveal unique genome wide patterns suggestive of selection at or below the
individual gene level. Further simulation studies will be performed to verify that SFP based
statistics accurately reproduce results from traditional nucleotide based methods (JIANG et al.
2005). Loci showing patterns of selection can be further validated in other population samples.
C) Reverse natural genetics resource. The resequencing data, of 384 A. thaliana genomes, can
be scanned for natural variation in genes of interest. This provides the Arabidopsis community
with a natural “change of function” catalogue of all genes that can be mined for phenotypic
variation. In particular, small and rare potential deletions can be identified within and between
genes that may be responsible for QTL (WERNER et al. 2005).
D) Algorithm development. New methods for analysis of gene expression, such as empirical
Bayes, will be incorporated into SFP detection. In addition, methods will be developed based on
Hidden Markov Models (HMMs) to account for insertion/deletion polymorphisms that affect several
features and to consider linkage disequilibrium in haplotype blocks. Finally, evolutionary models
will be developed through simulation and empirical sequence studies to define proper criteria for
using SFPs to measure diversity, selection and recombination rates (JIANG et al. 2005).
Improvements made to SFP detection/genotyping will be broadly applicable to other systems. All
2
RO1 GM073822 Principal Investigator/ Program Director: Borevitz, Justin
methods will be incorporated into the public open source Bioconductor (www.bioconductor.org)
packages.
Aim2: Genetic variation across seasonal and geographic natural environments.
Quantitative variation in flowering time will be measured across two seasonal (Fall and Spring) and
two geographic (latitudes of Spain and Sweden) environments encompassing the native range of the
species. Growth chamber conditions will mimic field settings by cycling temperature, light color, light
intensity, and day length, that change throughout the day and the “season”. Humidity will also be set
to mimic monthly regional averages. These conditions recapitulate the predictable seasonal
environmental cues and avoid the noise inherit to real field conditions. This and future community
quantitative data will be used for genome wide linkage disequilibrium quantitative trait locus (QTL)
scans. Population structure will first be accounted for using STRUCTURE. (FALUSH et al. 2003;
THORNSBERRY et al. 2001). The haplotype and quantitative data sets will provide a test bed for
development of association mapping methods. We will take advantage of methods recently
developed based on coalescent models for identifying QTL (HAGENBLAD et al. 2004; ZOLLNER and
PRITCHARD 2005). Here the ancestral recombination graph is inferred from the haplotype data and the
joint likelihood is evaluated across the graph, summing over the likelihood at each branch on the tree.
Ultimately the whole genome will be scanned for loci with this approach. Permutations of the
phenotype with genotype will be used to set experiment wise thresholds for detection that will account
for the large number of tests performed and other specifics of the experiment including haplotype
calling from SFP resequencing data, as well as adjust for any residual population stratification not
captured by STRUCTURE.
Aim3: Confirming loci and determining the molecular genetic basis of QTL.
To confirm associations identified through linkage disequilibrium mapping, and to revel the precise
genetic changes responsible for natural phenotypic variation, several strategies will be taken.
A) eXtreme Array Mapping (XAM) involves selection of accessions with different functional
haplotypes at the QTL. Genetic crosses are made and segregating variation is pooled according
to extreme phenotypes then mapped via bulk segregant analysis on arrays. Our recent studies
show several crosses can be quickly analyzed (WERNER et al. 2005; WOLYN et al. 2004), after
which fine scale recombination mapping can be performed if necessary with the aid of array
genotyping. Small effect associations can be confirmed by independent genotyping of many
progeny with markers at the candidate locus.
B) Candidate gene approach. This can be performed directly when association mapping
reveals a small interval or after recombination mapping has narrowed the region. Knock out
lines for all genes in the interval can be screened for quantitative phenotypes and be used for
quantitative complementation tests. Gene expression profiles will be obtained contrasting the
particular environments and QTL genotypes using pools of lines or isogenic pairs. Furthermore,
candidate genes will be investigated using public gene expression atlas data. Array hybridization
variation, from the haplotype map in Aim1 will suggest candidate genes (WERNER et al. 2005).
Finally, direct sequencing and transgenic complementation will be used to confirm that a QTL
have been cloned. This is especially important when functional alleles are not present in the
reference strain.
B. Background and Significance
With the completion of genome sequences from many organisms, attention is now focusing on
variation in the sequence and how this contributes to phenotypic diversity. It is now common to
determine the specific changes responsible for simple Mendelian traits in most organisms. As the era
3
RO1 GM073822 Principal Investigator/ Program Director: Borevitz, Justin
Figure 1. Haplotype block density. From (WALL
and PRITCHARD 2003) fig4. Four independent
simulations with recombination hotspots shown at
the bottom are shown. The first row estimates
haplotype blocks under experimental conditions of
sample size ~50 and SNP density of 6kb (GABRIEL
et al. 2002). The second and 4th rows are 8X
sample size, while the third and forth rows are 8X
in SNP density. Clearly the SNP density is a
major factor to reveal small haplotype blocks.
SFP resequencing would be especially
advantageous in this regard.
of single gene analysis fades, the next stage will be to determine the genetic basis of complex,
multigenic traits. Of particular interest is the genetic basis underlying common human diseases, the
genetic basis of adaptation, or the genes underling hidden genetic variation. Unlike Mendelian traits,
determining the genetic nature of complex traits is difficult since the overall phenotype is the result of
the environment, many genes, and interaction of genes with unique environments. To detect multiple
genes with small effects that may be dependant on the genetic background and/or the environment, a
statistical linkage is sought between the quantitative measure of the complex trait and a region of the
chromosome (MACKAY 2001). This is has been quite successful in model organisms, where large
experimental mapping populations exist and environments can be fixed under constant conditions;
however, this is much more difficult in humans or other organisms with slow generation times that
cannot be grown in the lab. Association mapping uses outbred populations, whereby many
“unrelated” individuals can be selected at similar ages and from similar environments (FLINT-GARCIA
et al. 2003; GOLDSTEIN and WEALE 2001). Association studies, with matched case (diseased) and
control samples, would be more powerful than linkage analysis to determine the location of complex
disease-causing genes, if all variants could
be identified and typed in a large population
(RISCH and MERIKANGAS 1996). At the moment
this is only feasible in some systems such as
microorganisms and now nearly so in
Arabidopsis (see below). In the absence of
complete resequencing, hope lies in exploiting
the nonrandom nature of sequence variation,
or linkage disequilibrium (LD). With LD, the
genotype at one marker is correlated with the
genotype at another. This relaxes the
requirement that each marker be typed
individually with high accuracy. The extent to
which this is true depends on the extent of LD.
Furthermore LD creates regions of SNPs that
are highly correlated, known as haplotype
blocks.
Haplotype map and marker density
The human haplotype map (HapMap) hopes
to determine all ancestral chromosome
blocks, which may be separated by
recombination hotspots, upon which
association (LD) mapping will be used to
identify regions responsible for complex
disease (INTERNATIONAL_HAPMAP_PROJECT
2003). Currently the human HapMap has >1
million SNPs typed from 4 populations
(http://www.hapmap.org/ ) (HINDS et al. 2005).
Phase of the heterozygous outbred genotypes
are being determined by typing, trios, mother,
father and child. This is not needed in
Arabidopsis since they exist as inbred lines.
How well will this study design cover
the genome with haplotype blocks? A recent
review uses simulations under models made
4
RO1 GM073822 Principal Investigator/ Program Director: Borevitz, Justin
from actual data to answer this question. With the current protocol and modest recombination
hotspots, ~27% of the genome will be covered (WALL and PRITCHARD 2003). Increasing the marker
density by 8 fold will capture about 71% of the genome, whereas increasing sample size has minimal
effect (Figure 1). With single marker technologies this results in an 8 fold increase in cost, however
highly parallel methods (e.g. (HINDS et al. 2005; PATIL et al. 2001) and SFP resequencing arrays,
discussed below) are ideally suited.
Haplotype association analysis marks the transition away from a single marker association
approach and is much more powerful for association mapping (LI and STEPHENS 2003) given good
haplotype coverage. Rather than testing single changes in isolation, haplotypes are now used as the
unit of inheritance. This has more biological significance because, no variant is an island; the local
genetic context needs to be considered. Identification of common haplotypes will be especially
important for mapping common traits when they are caused by common mutations. For rare alleles
typing of the causal variant may be required in specific samples where the allele may be at relatively
higher frequency.
The marker resolution required for the successful creation of a haplotype map depends heavily
on the extent of LD. Studies to determine the extent of LD have been performed in humans,
Drosophila, and Arabidopsis. Studies in humans have determined that the genome may consist of
blocks (10-100kb) with limited haplotype diversity separated by recombination hotspots (DAWSON et
al. 2002; GABRIEL et al. 2002; HINDS et al. 2005; PATIL et al. 2001; PHILLIPS et al. 2003; REICH et al.
2001). Blocks of extensive LD have not been seen in Drosophila. Here, recombination is much
more rampant, reducing LD to a scale of 100s of base pairs (LONG et al. 1998). Theoretical and
recently our empirical studies have shown that LD in Arabidopsis is much more extensive [on the
scale of humans, 10-100kb (NORDBORG 2000; NORDBORG et al. 2002; NORDBORG et al. 2005)].
Haplotypes are clearly defined due to a major reduction in effective recombination caused by selfing,
because most recombination events are between homozygous loci and chromosome segments are
not broken up. The effective mutation rate however is only partially affected by selfing (FLINT-GARCIA
et al. 2003; NORDBORG 2000). The resulting high ratio of diversity to recombination rate makes LD
mapping in Arabidopsis particularly powerful, because haplotype blocks are more extensive and
well defined.
The empirical studies in humans, Drosophila, and Arabidopsis also highlight the unexpected
large variance in the extent of LD. Not all markers at close distances show high levels of LD. As a
result, placing markers at a uniform density, as determined by the mean extent of LD, will not be that
useful in determining the majority of haplotypes (GOLDSTEIN and WEALE 2001). Determining the
marker resolution required to identify all common haplotypes in an organism is difficult; certainly a
high density will be required initially [(KRUGLYAK 1999) and (GABRIEL et al. 2002) suggested 1 SNP
every 3-10kb for humans; 300,000 – 1,000,000 markers], after which a smaller set could be defined if
necessary. When scanning at low density for haplotype blocks, if the allele frequency at the causal
variant does not match with the frequency of the haplotype then selecting SNP tagged haplotypes for
associations will have decreased power (ZHANG et al. 2004).
Array Genotyping (BOREVITZ et al. 2003)
Given the enormity of the task in creating a HapMap, what technique is suitable to identify and
genotype markers at high enough resolution to determine all the haplotypes? Originally, in
collaboration with Elizabeth Winzeler, I have developed methods for parallel discovery and
genotyping of tens of thousands of Single Feature Polymorphisms (SFPs) using Affymetrix
GeneChips. The technique, originally developed in yeast (WINZELER et al. 1998), has now been
extended to an organism that has a 10 times larger genome. By hybridizing randomly labeled
genomic DNA from different strains, using biological replicates, each oligonucleotide feature is tested
statistically for a difference in hybridization intensity, indicative of a polymorphism (Figure 2). To
control for the specifics of the experiment and the immense number of statistical tests, the
5
RO1 GM073822 Principal Investigator/ Program Director: Borevitz, Justin
Whole Genome Array Genotyping
Col/Ler merged
Col Ler
Potential deletions Single Feature
Polymorphisms
(SFPs)
Figure 2. Genomic DNA hybridization to
oligonucleotide arrays. A comparison of two accessions
reveals hybridization differences at a fraction of features
(squares) as either red or green for greater intensity of one
strain or the other.
permutation distribution was used to
determine a 5% false discovery rate
(FDR) (TUSHER et al. 2001). Ler
sequence available in databases
was used to independently
determine a 3% FDR and that the
sensitivity was a high as 85%
(BOREVITZ et al. 2003).
Approximately 4% of features are
called SFPs when a single
Arabidopsis accession is compared
to the reference strain. Only
markers where the reference
genome has a greater hybridization
signal are used, thereby insuring the
correct physical location of each
SFP. In addition, only features that
map to a single unique region in the
reference genome are considered,
thereby avoiding duplication.
Potential deletion polymorphisms
were identified as clusters of SFPs
and were prevalent in transposonlike genes, disease resistance
genes, and genes controlling
secondary metabolism (BOREVITZ et
al. 2003). SFPs in coding regions and potential deletions identify candidate genes for QTL (WERNER
et al. 2005).
We further tested the SFP markers by segregation analysis in 2 experiments. First a
recombinant inbred line was genotyped using a single hybridization. Here the thousands of SFPs
could clearly distinguish the parental genotypes and delimit recombination events (see figure 4 of
(BOREVITZ et al. 2003)). Second we performed a mapping experiment by bulking either wild-type or
mutant segregating plants and hybridizing labeled DNA from each bulk pool to an expression array.
The allele frequency differences between the pools at linked SFPs can be accurately estimated from
that array hybridization data. SFPs near the mutation (in the Ler background) are enriched for Ler
alleles, while the wild type pool is enriched for Col alleles. Unlinked loci are at approximately equal
frequencies in each pool. To date at least 4 labs have been successful in mapping more than 10
mutations. Four loci that mapped to candidate genes were confirmed to have sequence changes
revealing new alleles of known genes (HAZEN et al. 2005). This empirical work agrees with
simulations showing the mapping accuracy to be <7cM or +-2Mb. Fast neutron deletions were also readily identified using array hybridization and could also be detected in heterozygous form (HAZEN et al. 2005). This method has attracted widespread attention both within and outside the plant community. I recently published a methods paper to help distribute the technique (BOREVITZ 2005). eXtreme Array Mapping For this proposal, the next step was to extend array mapping to quantitative traits, now called eXtreme Array Mapping (XAM) (WOLYN et al. 2004) (WERNER et al. 2005). We now have 3 published examples where major Quantitative Trait Loci were identified in pools of extreme lines. To map seedling light response the tallest 15 recombinant inbred lines (RILs) were pooled and compared against the shortest 15 RILs. Differences in allele frequencies between the pools reveled the location 6 RO1 GM073822 Principal Investigator/ Program Director: Borevitz, Justin of the QTL (WOLYN et al. 2004). A second example contrasted pools of the 50 earliest vs 50 latest flowering plants from a pool of 561 F2 plants. A similar 3rd experiment contrasted 65 early vs 65 from 330 F2s. All QTL identified by XAM were confirmed by traditional linkage mapping. In an example from humans, pooling genomic DNA samples followed by quantitative genotyping with array hybridization was recently shown to be effective at identifying cholesteryl ester transfer protein (CETP) as a candidate gene for controlling HDL cholesterol levels (HINDS et al. 2004). Identification of by Flowering QTL by array hybridization. We have mapped a flowering time QTL in the Nd-1/Col-0 RIL set in both long and short days with additional genotyping at 79 SNP markers (WERNER et al. 2005). A major QTL, FLOWERING1, on the bottom of chromosome 1 was subsequently fine mapped to 140kb. To identify candidate genes for phenotypic variation we looked at the pattern of genomic DNA hybridization on expression arrays. A comparison of hybridization patterns between Col and Nd-1 identified a potential deletion in a MADS box gene FLM (RATCLIFFE et al. 2001; SCORTECCI et al. 2001). PCR and subsequent sequencing revealed that indeed Nd-1 has a 6,817 bp deletion that completely removes FLM. When FLM-Col was transformed into a near isogenic line containing the flm-Nd allele, flowering time was restored. Interestingly, the deleted sequence is flanked in the reference wild type, Col-0, by a 7 bp repeat, GTATAAT, of which only one copy remains in Nd-1. Here array hybridization rapidly revealed the causative mutation. SFP identification in the transcriptome (ROSTOKS et al. 2005) SFP detection in large genomes is difficult due to the reduced signal to noise ratio. The barley genome is ~6Gb (2X humans, 50X Arabidopsis) and was a test case to see how far whole genome labeling and SFP detection could be pushed. Genomic DNA was randomly labeled and hybridized from 3 barley genotypes in triplicate. Here only 1090 SFPs were identified at a 22% False Discovery rate set by permutations (ROSTOKS et al. 2005). Although successful, additional replication and/or amplification of a subset of genomic DNA should improve signal to noise for SFP identification in large genome organisms. Another approach is to use the transcriptome as a reduced representation of a large genome for SFP identification if overall gene expression variation can be accounted for. Barley gene expression data was available from two genotypes across six tissues in triplicate. A linear model was fit for each gene to account for overall gene expression variation between genotypes and tissues. The final term in the model looked for a feature by genotype interaction indicating the hybridization intensity of an individual 25mer depended on the genotype of the RNA sample. This analysis revealed 10,504 SFPs at a low 0.1% FDR set by permutations. Sequencing and EST data covering 2601 features, revealed ~60% sensitivity even when only single tissues were compared (ROSTOKS et al. 2005). SFP genotyping from expression data was also recently shown to be effective in yeast (RONALD et al. 2005). SFP identification from transcriptome data can identify polymorphisms representing DNA sequencing polymorphism as well as alternative transcripts when individual 25mer features are detected with differential hybridization. To distinguish between these two, SFPs from the transcriptome are compared with SFPs from genomic DNA. This allows one to determine the extent of alternative splicing between genotypes (a completely outstanding question well addressed on the new AtTILE1 array). Preliminary results show that this is working. Expression profiling for candidate gene identification A powerful approach to the rapid identification of QTL genes is the union of expression and mapping studies. From the handful of QTL that have been cloned it appears that several are caused differential gene expression. The fw2.2 allele, causing large fruit size in tomato, reaches peak levels of expression one week later than plants carrying the small-fruited allele (Cong et al. 2002). At a locus controlling apical dominance in maize, the maize allele of teosinte branched1 is expressed at much higher levels than the teosinte allele (Wang et al. 1999). Even if the causative change is not 7 RO1 GM073822 Principal Investigator/ Program Director: Borevitz, Justin regulatory, i.e. a coding deletion or amino acid change, often the transcript is destabilized, which can be measured by hybridizing RNA to a GeneChip. One example is rice Hd1, a major QTL controlling flowering in response to photoperiod, where allelic variation results in differences in message length. This would be identifiable on whole genome tiling arrays (Yano et al. 2000). The general approach has been termed transcription based cloning (MITRA et al. 2004). We have used this approach to identify a candied gene for the LIGHT1 QTL, as well as potential downstream targets (see preliminary results). Figure 3. Expected number of SFPs. There is a linear relationship between nucleotide diversity, theta and the expected number of SFPs under several models. Circles denote the estimated values from simulations. Theoretical lines are drawn according to formula (6) in (JIANG et al. 2005). Four cases: independent calling with (sensitivity, specificity) as (0.34, 1.0 red), or dependent calling (0.34, 1.0 black), ideal case (1.0, 1.0 green), or lower SFP threshold (0.68, 0.75 blue). SFPs for population genetic analysis In a recent theory paper we develop statistical methods for inferring evolutionary parameters from SFP data (JIANG et al. 2005). SFPs can are thought of as SNPs, but with different underlying properties. Specifically, the form of the final data is the same in both cases, there are two allelic states. This suggests it may be possible to take existing SNP-based methodologies and adapt them for use on SFP data. The approach is based on rejection methods where one begins by calculating informative summary statistics for a data set of interest. Here the population genetic measure of mutation rate (θ) and recombination rate (ρ). Data is then simulated under a variety of parameter values, taking note of parameter values that lead the simulated data look like the real data. This similarity is measured via the use of summary statistics. The results are used to construct posterior distributions for the parameters of interest. For an example application of these methods see (PADHUKASAHASRAM et al. 2004; TAVARE et al. 1997). Jiang et al. show that a rejection method based upon a summary statistic which records the number of sites at which an SFP is observed, has good power to estimate θ. The expectation of the observed number of SFPs depends almost linearly on θ for a variety of beliefs about sensitivity and specificity of the SFP calling procedure (Figure 3). Of more interest for haplotype determination is the estimation of recombination rate, ρ, here tested using the rejection method and maximum likelihood estimates for two locus sampling. They show that the performance of the rejection method decreases as sensitivity and specificity decreases. The rate of information loss is crucially dependent on the notion of independence versus dependence (across accessions) when calling SFPs. If calling errors at a given SFP location are highly correlated the loss of information is minimal. This is generally the case in practice since when 25mer features behave well, (good hybridization and good discrimination) they do so across all accessions tested. Preliminary data shows that some SFPs have very good correlations with sequence data, while other are less so. If, however, errors are independent, information loss is greater. This property will help guide us when designing improved SFP-calling procedures, and will enable us to maximize the information attainable for the parameters of interest. A clear first step is to perform F tests across accessions for each feature so the calls are explicitly dependant. Finally, Jiang et al use adaptations 8 RO1 GM073822 Principal Investigator/ Program Director: Borevitz, Justin of Fearnhead 2003 and Hudson 2001 to derive theoretical results for the properties of SFPs. They then use these results as the basis of a further method to estimate recombination rate from SFP data. These results show SFPs perform as well as SNPs spaced 1 every 10kb to estimate ρ and often have smaller error rates under realistic experimental designs Figure 4 Arabidopsis Phylogeny A Phylogenetic tree, made from polymorphisms identified from over 1000 genome wide sequencing reactions (>15,000 SNPs), showing population structure in a sample
chosen to represent local, regional, and worldwide collections
(Hhttp://walnut.usc.eduH )
Population Structure in
a regional collection
Figure 4 shows a
phylogeny of 96 A.
thaliana strains that were
selected for the 2010
diversity study from
specific regional locations
(NORDBORG et al. 2005).
The tree is supported by
sequence variation at
over 1000 loci making
deep population structure
inferences possible. What
is striking is that there is
ample variation within
regions in addition to the
expected population
structure. For example,
in accessions collected
from the USA, Pna-10,
Knox-10, RRS-10 are
basically identical siblings
from different cities in
Indiana and Michigan,
while Knox-18 is as
different from Knox-10 or
RRS-7 (being from the
same fields) as they are
to other European strains.
This is an extreme
example, however it is
clear that there are many
deep branches in
9
RO1 GM073822 Principal Investigator/ Program Director: Borevitz, Justin
England, Germany, and especially the Balkans. This suggests that a wide screening of all available
accessions to first look for identical siblings, then with a deeper SNP panel, can identify many of the
short branches or clear relatedness. Subsequently a core collection could be identified from a couple
of generally distantly related samples showing little stratification.
LD mapping of known flowering time loci: test case (HAGENBLAD et al. 2004)
A study of natural variation in flowering time among hundreds of accessions was performed through a
collaboration between the Salk Institute and USC. Two candidate loci, FRI and FLC, were
investigated for patterns of polymorphism at flanking intervals and LD fine mapping was performed.
The results showed that a novel Bayesian algorithm could account for allelic heterogeneity and
delimit FRI to a 30kb region revealing two independent loss of function haplotypes. Single marker
analysis was much less precise. Both marker density and the amount of fine recombination in the
population were limiting for further fine mapping at FRI and FLC. This study provides an important
analytical and empirical foundation for the proposed whole genome scan studies, including
consideration of the effects of population structure.
Association mapping by estimation of the Ancestral Recombination Graph
Another approach to association mapping tested relies on our recent studies which estimate the
ancestral recombination graph (Figure 5.). Here the joint tasks of identifying linkage and localizing it
to a fine chromosomal region is divided into two parts (ZOLLNER and PRITCHARD 2005). First the
haplotype data is used to estimate coalescent genealogies of the sampled inbred (haploid)
chromosomes (Figure 5, trees on the right). Then associations are performed testing each branch. A
joint association statistic is made by summing the association result across all the nodes. Finally
permutations are performed to assess significance.
SFP resequencing for whole genome association studies
The SFP genotyping method is a unique
balance between whole genome
resequencing and selective SNP
genotyping. When sequence variation is
low, typical for within species studies, little
is gained from complete resequencing
and certainly there is substantial cost
associated with complete resequencing of
hundreds or thousands of different
individuals (HINDS et al. 2005). SNP
genotyping, on the other hand, looks at
regularly spaced predefined intervals and
rarely identifies the causative
polymorphism. In addition there may be
ascertainment bias when genotyping
known polymorphisms in other
populations (AKEY et al. 2003). Thus high
density SFP genotyping is an ideal
compromise for identifying haplotype
blocks in LD, and for potentially revealing
causative changes such as rare small
deletions. Greater than ½ of the whole
genome can be interrogated with a single
hybridization and many individuals can be
10
Figure 5. Ancestral Recombination Graph. Each
chromosomal fragment (haplotype) has an
independent evolutionary history owing to
recombination such that regions have separate
ancestries. This results in different trees for physically
linked intervals.
RO1 GM073822 Principal Investigator/ Program Director: Borevitz, Justin
screened through additional hybridizations.
Knowledge of the exact bp change(s) is not
needed, rather we only need a way to
discriminate the different alleles and thus
haplotypes.
screened through additional hybridizations.
Knowledge of the exact bp change(s) is not
needed, rather we only need a way to
discriminate the different alleles and thus
haplotypes.
11
Figure 6. Images of A. thaliana haplotypes in
false color. Each column represents a unique
hybridization (accessions done in triplicate). Each
row is an ordered feature on the array
corresponding to a ~500kb region on chromosome
- SFPs are a consistent red color (low
hybridization) across the 3 replicates. Haplotype
patterns can be clearly seen, for example Bay and
Lz have a very similar pattern, Cvi and Nd match
the reference Col haplotype. Ler and Shah
correspond to a third haplotype, while Kas may be a
4th. Non polymorphic features (rows) are
intermediate orange color across all accessions and
are included for spacing. This haplotype pattern
changes as one scrolls down the chromosome,
reflecting ancestral recombination events.
H/haplotype/hapsfpchr1.pd
fH
C. Preliminary Studies
To assess the feasibility of creating a
HapMap with array genotyping, and to
investigate patterns of variation throughout
the genome, we have begun a preliminary
investigation of SFP variation across 20 A.
thaliana accessions using the standard
gene expression array, which has ~200,000
unique 25mer features. In this study 2-3
replicates of each accession were
compared against matched replicates of the
Columbia reference strain. After spatial
correction (BOREVITZ et al. 2003) and
quantile normalization (BOLSTAD et al.
2003), modified t-tests were applied to call
SFPs with significantly lower hybridization
than the reference. Calls with higher
intensity are removed as duplication
polymorphisms have unknown physical
location. Figure 6 shows an example of 8
accessionsin false color (3 replicates in
columns). As described in the caption,
haplotype patterns are clearly seen.
To assess the feasibility of creating a
HapMap with array genotyping, and to
investigate patterns of variation throughout
the genome, we have begun a preliminary
investigation of SFP variation across 20 A.
thaliana accessions using the standard
gene expression array, which has ~200,000
unique 25mer features. In this study 2-3
replicates of each accession were
compared against matched replicates of the
Columbia reference strain. After spatial
correction (BOREVITZ et al. 2003) and
quantile normalization (BOLSTAD et al.
2003), modified t-tests were applied to call
SFPs with significantly lower hybridization
than the reference. Calls with higher
intensity are removed as duplication
polymorphisms have unknown physical
location. Figure 6 shows an example of 8
accessionsin false color (3 replicates in
columns). As described in the caption,
haplotype patterns are clearly seen.
SFP SNP Total FPR FDR Sensitivity
bay 44 61 1375 0.8% 25.0% 54.1%
bur 47 57 1320 1.1% 29.8% 57.9%
cvi 69 92 1325 1.2% 21.7% 58.7%
ler 41 51 1466 0.6% 22.0% 62.7%
lz 37 40 1441 0.5% 18.9% 75.0%
mr 67 87 1191 1.1% 17.9% 63.2%
mt 46 48 1413 0.9% 26.1% 70.8%
sorbo 37 53 1317 0.9% 29.7% 49.1%
ws 29 47 1369 0.3% 13.8% 53.2%
Table 1. Correspondence of SFP genotyping and
SNPs identified by sequencing. SFPs have a low
false positive rate and good sensitivity at the chosen
threshold of >50,000 total SFPs. Since SFPs are
quantitative, other thresholds can be chosen.
Table 1. Correspondence of SFP genotyping and
SNPs identified by sequencing. SFPs have a low
false positive rate and good sensitivity at the chosen
threshold of >50,000 total SFPs. Since SFPs are
quantitative, other thresholds can be chosen.
To determine the number of SFPs at
different false discovery rates, permutation
testing was applied (TUSHER et al. 2001).
Greater than 50,000 SFPs can be called at
an experiment-wise 1% False Discovery
Rate (FDR) across the 20 accessions,
including ~30,000 non-singleton SFPs.
To independently determine error rates,
and to see how many known SNPs could
be detected (sensitivity), we turned to
sequence data from the 2010 A. thaliana
diversity study (http://walnut.usc.edu
To determine the number of SFPs at
different false discovery rates, permutation
testing was applied (TUSHER et al. 2001).
Greater than 50,000 SFPs can be called at
an experiment-wise 1% False Discovery
Rate (FDR) across the 20 accessions,
including ~30,000 non-singleton SFPs.
To independently determine error rates,
and to see how many known SNPs could
be detected (sensitivity), we turned to
sequence data from the 2010 A. thaliana
diversity study (http://walnut.usc.edu)
(NORDBORG et al. 2005). Table 1 shows
the false positive rate (potential miscall
rate at each feature), the false discovery
rate (potential miscalls of the total
features identified) and the sensitivity
(number of known SNPs also identified
at SFPs). Missing a known sequence
polymorphism (low sensitivity) can be
RO1 GM073822 Principal Investigator/ Program Director: Borevitz, Justin
attributed to a poorly hybridizing feature, or often to a polymorphism that resides at the edge of the
25mer (BOREVITZ 2005; RONALD et al. 2005; ROSTOKS et al. 2005). Lowering the threshold and/or
increasing the number of replicates improves the sensitivity. Potential miscalls of SFPs that do not
contain a sequence polymorphisms include statistical false positives as well as true markers that are
likely the result of changes just outside the 25mer where alleles are differentially labeled. In the later
case SFPs will be informative about haplotype patterns. Another important issue is discrimination of
multiple SFP alleles. Different SNPs in the same 25mer feature could be detected as SFPs but could
not be distinguished as different genotypes. Sequence data shows that this happens rarely. Of the
406 features with known polymorphisms 331 (82%) are biallelic just like SNPs, 63 are triallelic (16%),
and 12 (3%) reside on a micro-satellite with >3 alleles. The entire SFP collection has been made
available in a web-searchable format allowing users to search genomic regions or genes for natural
variation (http://natural.salk.edu/cgi-bin/sfp ).
We next turned to patterns of variation across the genome. SFP diversity can be measured in
two common ways. One is related to total diversity, Watterson’s θ (WATTERSON 1975). We calculated
the percent of polymorphic SFPs in 50kb sliding windows along the genome. Figure 7 shows that this
pattern is highly variable; however gross chromosomal features are apparent. The centromeres on
all chromosomes are highly variable as well as region at the top of chromosome 4 which corresponds
to the heterochromatic knob (FRANSZ et al. 2000). Thus we see a negative correlation between
polymorphism and recombination rate. The prevalence of insertion/deletion polymorphisms near the
centromere may partially explain this pattern in our data. To create a null hypothesis for the
positional patterns of variation, SFP genotypes were randomly shuffled with respect to position along
the chromosome. Diversity was then recalculated in 50kb sliding windows along the genome. This
shuffled data set can set limits under the null hypothesis that there is no positional pattern to the
variation seen (figure 7 and 8, red horizontal lines, figure 9 vertical lines).
Figure 7. Patterns of variation across the genome as seen
from SFP genotyping. The centromeres on all 5 chromosomes,
and a bump at the bottom of chromosome 1 and 4, show
increased levels of variation. The red line is smoothed average,
the blue line is the chromosome mean, and black line is the
diversity in 50kb sliding windows calculated at 5kb steps.
We next looked in detail at particular chromosome 5 region with a high level of diversity (Figure
8). Here two measures of diversity are shown calculated in the 50kb sliding windows, total diversity θ
(black line), and pair-wise diversity (TAJIMA 1983) π (red line). In order to compare the two diversity
measures using SFP data
,both were scaled to a
genome-wide mean of 0 and
standard deviation of 1. There
are 3 peaks shown in figure 8
that are in the upper 2.5% tail
of the chromosome-wide
diversity distribution, they
exceed the horizontal black
line. This suggests that this
locus is evolving much faster
then other regions in the
genome. A comparison of the
two measures of diversity
reveals different patterns of
selection. θ is sensitive to rare
changes, while π is greater
when there are a few common
haplotypes. The difference
between the two measures is
analogous to Tajima’s D
statistic, a test for selection
(TAJIMA 1989). Again we
12
RO1 GM073822 Principal Investigator/ Program Director: Borevitz, Justin
Figure 8. Diversity at the RPS4 locus. The RPS4
family of duplicated R genes is shown in blue. Other
genes are shown with the thick black line. The thin
black line traces total diversity, while the red line traces
average pair-wise diversity in 50kb sliding windows
across this region. Horizontal thresholds represent 95%
of the actual diversity along the chromosome, in black,
or 95% of the diversity under the null hypothesis in red.
compare a given region to the genomewide empirical distribution. Figure 9
shows the pattern of variation in
Tajima’s D-like statistic at the RPS4
locus. Two patterns of selection can be
identified that are rare in the genome (< 5%). In regions that co-localize with the disease resistance (R) genes (shown in blue) θ is greater than π, yielding a negative Tajima’s D-like statistic. This rare patters suggests diversifying selection with an unusual excess of rare mutations. At an adjacent locus (to the right) π is greater thus Tajima’s D-like statistic is positive, indicating that balancing or frequency dependant selection may have maintained old haplotypes. This rare genomic pattern of variation suggests this locus of unknown genes may be functionally important and has played a role in past evolution. Further functional tests may be warranted including sequence diversity studies in additional populations. The genome can be scanned for patterns suggestive of selection and this can reveal further clues about the evolutionary importance of known genes or suggest functions for unknown genes (data available at /sfp/ath1sfp.html ). There are 149 genes in the Arabidopsis genome which have a protein sequence similar to known pathogen disease Resistance (R) genes (MEYERS et al. 2003). We choose another family of 148 bHLH transcription factors (http://arabidopsis.med.ohiostate.edu/) as a control. Figure 10 shows the distribution of Tajima’s D-like scores for R genes and bHLH genes. There are 30 and 29, R genes that are reside in the lower and upper tails of the genome wide distribution. In contrast, the bHLH family contained only 4 and 4 genes in the lower and upper tails. Balancing selection has been inferred by sequencing studies at three R gene loci (MAURICIO et al. 2003; STAHL et al. 1999; TIAN et al. 2002) and may be due to a cost associated with resistance (TIAN et al. 2003). However diversifying selection is also known and may be due 13 Figure 9. Patterns of selection at the RPS4 locus. The thin black line traces the difference between total variation and pair-wise variation, similar to Tajimas’ D statistic. Negative values indicate diversifying selection, while positive values indicate balancing selection. Horizontal lines are as in Figure 8. RO1 GM073822 Principal Investigator/ Program Director: Borevitz, Justin to an evolutionary arms race of competing pathogen virulence and plant resistance (BERGELSON et al. 2001). We reveal rare genomic patterns suggestive of both types of selective forces. These R genes have been, or are perhaps still active while others may be pseudogenes. Another locus with a rare pattern suggestive of balancing selection was the self incompatibility locus (S-locus) on chromosome 1, known in other species to be under heavy frequency dependant selection. (/sfp/ath1sfp.html ). The S-locus may have recently lost function in A. thaliana, allowing it to become a selfer, however the rare signature suggesting selection seems to still remain (NASRALLAH et al. 2002). (-1,-0.8] (-0.6,-0.4] (-0.2,0] (0.2,0.4] (0.6,0.8] Selection Tajima’s D like statistic frequency 0 10 20 30 40 50 60 70 Rgenes bHLH Figure 10. Distribution of Tajima’s D like statistics for R genes and bHLH genes. The vertical lines contain 95% of the calculated statistics under the null hypothesis. Use of Expression Profiling for Candidate Gene Identification. We present preliminary evidence here as an example of how gene expression profiling could be used to help identify QTL for Aim3. The LIGHT1 locus was detected as QTL controlling seedling elongation light response (BOREVITZ et al. 2002). To investigate the extent of gene expression differences between accessions at the young seedling stage, and to identify candidates for the LIGHT1 QTL, we analyzed the transcription profile of the parent lines Ler, Cvi, and the LIGHT1-Near Isogenic Line (LIGHT1-NIL) (BOREVITZ et al. 2002). RNA from three independent biological replicates of each genotype was hybridized to expression arrays that contained about 1/3 of annotated Arabidopsis genes (8,247). At this stage differences in hybridization intensity could be due to differences in true gene expression or to DNA polymorphisms. However, either cause could reveal candidate genes. The first question was, how many of the genes that were differentially “expressed” between Ler and Cvi mapped to the LIGHT1 QTL region? Multiple t-tests were performed and significance was evaluated by comparison with the permutation distribution (TUSHER et al. 2001). 166 genes were detected between Ler and Cvi at an 18% FDR. Of these, 7 fell into the LIGHT1 QTL region, which extends over about 4 Mb. This initial array, however only contains 271 out of the about 1,000 genes in the LIGHT1 QTL region. Odds were against finding the actual LIGHT1 gene but downstream targets may be identified. When comparing Ler to the LIGHT1 NIL, the number of differentially hybridizing genes was dramatically reduced, from 166 to 15, of which 6 fell into the QTL interval. All but one of these is the same as the ones discovered in the comparison between Ler and Cvi. Thus LIGHT1 was not controlling all of the 166 Cvi/Ler differences. 14 RO1 GM073822 Principal Investigator/ Program Director: Borevitz, Justin Figure 11. Downstream response candidate A) PAG1 is differentially expressed between Ler and Cvi..B) pag1 KO mutants (SALK 040211) have a pale phenotype. C and D) pag1 plants have long hypocotyls in red light. E and F) pag1 mutants are early flowering, similar to phyB mutants. We next used DNA hybridization to the same arrays to determine which changes were due to underlying polymorphisms and which were due to true expression changes, (BOREVITZ et al. 2003)(reviewed above). SFPs were identified and removed prior to re-analysis of gene expression. This comparison of Ler and Cvi identified 96 true gene expression differences at a 20% FDR. Further studies were performed, using new arrays and more resolved near isogenic lines, to reveal LIGHT1 candidates. Here, an unlinked gene that was truly differentially expressed was further investigated using KO lines. At2g24790, is a CONSTANS homologue we named PALE GREEN1 (PAG1) (Figure 11). pag1 mutants were identified as pale when grown in white light – a phenotype similar to phyb mutants. Indeed pag1 plants have elongated hypocotyls in red light as well as increased flowering time (Figure 11 C-F). pag1 does not map to any predicted QTL for light response. It is likely that transcription of PAG1 is controlled by Cvi x Ler LIGHT QTL and thus is part of the signaling cascade of natural variation in light response. As Cvi expresses lower levels of PAG1 and is less sensitive to light (as are pag1 mutants), we may have identified an important downstream component of light response variation between Cvi and Ler. We (now mostly Olivier Loudet (INRA) and Todd Michaels (Salk)) have followed the LIGHT1 QTL gene in detail and are very near to cloning it. I briefly present the work done as an example of the power of Arabidopsis genetics for identification of QTL that will be used in Aim3 of this study (BOREVITZ and CHORY 2004; BOREVITZ and NORDBORG 2003). Beyond the LD mapping and transcriptional analysis of LIGHT1 discussed, we have used recombination fine mapping to genetically define the interval to < 135kb (35 genes) by screening over 2000 F2 plants and progeny testing all recombinants. We have used array genotyping to precisely determine the narrow recombination breakpoints and to confirm the isogenic background. We have investigated genes in the region for insertion/deletion polymorphisms and for SFPs that may have functional consequences (WERNER et al. 2005). However, this region contained relatively few SFPs. Indeed, diversity levels at this locus are in the lowest 2.5% of the genome, suggesting a possible recent selective sweep. Subsequently, homozygous knock-out insertion mutants were available and screened for 22 genes in the interval. They did not reveal significant quantitative differences in light response, although one line KO At1g21600 showed a severe pale phenotype that was lethal after 10 days. Gene expression analysis has revealed 55 genes differentially expressed between the small introgression line and its isogenic counterpart, 1 of which maps to the narrow QTL region. This gene is also At1g21600. A QTL for circadian rhythm co-localizes with LIGHT1 (SWARUP et al. 1999) and transcriptome Atlas searching revealed At1g21600 is circadian regulated (Sam Hazen, personal communication). Throughout the candidate gene selection process, knowledge of the light signaling, flowering, and 15 RO1 GM073822 Principal Investigator/ Program Director: Borevitz, Justin circadian rhythm pathways was utilized. At1g21600 however is a single copy gene found only in plants with no homology to other characterized proteins. Sequencing variation among 96 accessions revealed that a single candidate amino acid change between Ler and Cvi was at low frequency but has independently mutated in other distantly related species. Final proof that LIGHT1 = At1g21600 is under way, including quantitative complementation tests, further fine mapping and transgenic studies. This example illustrates how the vast genomic resources available in Arabidopsis aid in cloning QTL. Linkage Disequilibrium Mapping at LIGHT1 and LIGHT2. After initial QTL mapping (BOREVITZ et al. 2002) we began studies to determine the extent of LD at both LIGHT1 and LIGHT2 and to more precisely delimit the QTL intervals (HAGENBLAD et al. 2004). At LIGHT1 we sequenced 27 fragments from 96 accessions, spanning 1 Mb either direction, and discovered 387 SNPs. At LIGHT2 we sequenced 11 fragments from the same 96 accessions, spanning 50kb north of PHYB to 200kb south of PHYB. Pair-wise LD (r2 correlation coefficients between markers) decays on the scale of ~100kb at the LIGHT1 locus and faster at PHYB. We are currently using Bayesian methods for LD fine mapping this region (HAGENBLAD et al. 2004). So far, pair-wise association tests are suggestive of our candidate genes. Since both LIGHT loci may to be at moderate frequency within A. thaliana (overlapping linkage detected in other RIL crosses, Kas x Col and Bay x Shah) we expect they are suitable real world test cases for the proposed methods of haplotype association mapping. SFPs from plastid genomes The Arabidopsis gene expression array contains 696 and 249 unique features matching the chloroplast and mitochondrial plastid genomes respectively. Testing for reproducible hybridization differences across the 14 accessions, revealed a 198 SFPs as singletons and 145 SFPs across multiple strains (20% false discovery rate set by permutations). Furthermore the Shahdara accession contained a deletion of 3 adjacent unknown open reading frames in its mitochondrial genome. SFP resequencing of both plastid genomes will be possible using whole genome tiling arrays (see below). D. Research Design and Methods Aim1: Determination of high density A. thaliana haplotype map. The first aim is the foundation for our proposal and will be a tremendous resource for the community. The majority of the experimental funds are sought here and prices are likely to decrease with time. The proposed technology gives a spectacular amount of data that is especially suited for our study. Whole genome oligonucleotide tiling arrays will be used for SFP resequencing of 384 A. thaliana genomes. SFP resequencing does not reveal the exact base pair changes between accessions, rather hybridization polymorphisms covering the entire genome. Thus SFPs behave like any genetic other marker, e.g. SNP, RFLP, or AFLP (BOREVITZ et al. 2003). They will capture over 50% of the total sequence variation (72% coverage *>70% sensitivity) and, because of LD, nearly all of the
haplotype structure at relatively little cost (~$1100 total with 2) allowing large sample sizes to be
assayed. The final data set will represent over 1 billion genotype tests.
Multi use, Arabidopsis whole genome tiling array (AtTILE1).
This array has been designed in collaboration with Joseph Ecker at the Salk Institute and Tom
Gingeras of Affymetrix, and is now available for early access (see accompanying email). We have
worked closely on array design issues over the past 2 years. AtTILE1 is a substantial improvement
over the initial tiling array sets, with better oligo selection on a single array (YAMADA et al. 2003). After
initial testing, AtTILE1 will be commercially available to the community for ~$400 per array. This
array has much higher density than is currently available and will contain 6.3 million features. The
current commercial Arabidopsis gene expression array contains ~200,000 Perfect Match 25mer
16
RO1 GM073822 Principal Investigator/ Program Director: Borevitz, Justin
features designed against predicted genes, while initial tiling arrays contained ~800,000 PM features
(YAMADA et al. 2003). Importantly, AtTILE1 covers nearly the entire genome at an average of <= 35 bp resolution and was designed without regard to potential coding regions. Thus, variation can be observed within and between coding regions. In addition, gene expression can be detected on either strand, revealing potential antisense transcription. Repetitive regions in the reference genome are not included, so that 25 bp oligo nucleotide features match to a single unique region in the genome. Partial multiple matches are also removed so that hybridization signal is specific. Gene families or newly duplicated loci, however, can often still be analyzed since intervening unique 25mers can distinguish repetitive regions. This specificity is a clear advantage of short oligonucleotide arrays. Finally 25mers were chosen to have good hybridization properties as new methods are now publicly available to accurately predict binding affinity (HEKSTRA et al. 2003; WU and IRIZARRY 2004; ZHANG et al. 2003). AtTILE1 array will be a comprehensive, vestal tool, appropriate for many studies, including for example very high density polymorphism detection (SFP resequencing), gene expression, gene discovery, alternative splicing, and antisense transcription. Integrating a wide range of genomic data on a single platform will also prove very powerful (BOREVITZ and ECKER 2004). Choice of A. thaliana accessions for SFP resequencing. This is an important consideration since the final core set will be used by many labs for Association mapping and gene discovery. Furthermore, methods advanced from this study may guide future work in other organisms. To reduce the effects of population structure that limit power for association mapping (FREEDMAN et al. 2004; MARCHINI et al. 2004) we will follow a 3-stage sampling approach. In addition this will catalogue the genetic diversity within the species and suggest demographic effects that have shaped the current population. 1460 accessions are currently available in the stock center (www.arabidopsis.org) and several hundred others have been recently collected. This includes additional populations from Northern and Southern Sweden (Magnus Nordborg), collections from the Iberian Peninsula (Carlos Alonso Blanco), Tajikistan (Olivier Loudet), Turkey and Caucasus (Barbara Schaal), and new Indiana and Michigan collections (Joy Bergelson and Justin Borevitz). Accessions collected in the same field or locality may be nearly identical siblings, given the often high selfing rate. However this is clearly not always the case (Figure 3) and (NORDBORG et al. 2005). 1) The first stage will be to screen all available lines (~2000) to identify siblings by genotyping ~20 moderate frequency SNP markers which can discern populations (high Fst). This will be done in collaboration with the Ohio State stock center so that future distributions will all be molecular tagged and there will be no discrepancies between labs, (see letter from Randy School). DNA from tissue collected at Ohio State will be extracted at UChicago using a 96 magnetic bead Kingfisher 96 robot. A DNA stock will be sent for SNP genotyping (likely at Genaissance Pharmaceuticals) and back to Ohio State if ample DNA remains for dilute stock distribution. Once siblings are identified a single line will be chosen to represent the group. 2) The reduced collection (perhaps 1000 lines) will be further genotyped with a panel of 384 moderate frequency SNP markers to determine gross population structure. These markers will be chosen from the 2010 diversity study such that they can capture known population structure, i.e. they have differentiated allele frequencies between populations (high Fst). The proposed SNP panel will be verified in their ability to resolve a similar tree to the one known from >15,000 SNPs (Figure 3). We expect shorter branches of closer ancestry to be easily
resolved. The resulting SNP identity matrix of up to 1000 by 1000 lines at 384 SNPs will be
clustered and tree pruning methods will be used to select subsamples that are roughly
equidistantly related with relatively deep ancestry. Thus close ancestry can also be removed,
resulting in a couple remaining nodes with deep branches of roughly equal length. This gives
the advantage that both general and sample specific traits can be mapped.
3) The final set of 384 accessions will be typed by SFP resequencing using AtTILE1. We expect
deep populations structure to be identified with the additional data and plan to account for it
17
RO1 GM073822 Principal Investigator/ Program Director: Borevitz, Justin
using STRUCTURE (FALUSH et al. 2003)
SFP Resequencing:
A preliminary small-scale investigation will be heavily replicated (minimum of 8 arrays for the
reference Col and test Ler strains) to determine power at different levels of replication. Next, SFP
marker discovery will be performed using 22 equally distantly related accessions (chosen from the
2010 set) using 4 replicate chips each. Based on current studies (above) and the distribution of allele
frequencies, this will identify >500,000 SFPs (~200bp resolution). Sequence information from > 1000
loci is available from these strains to optimize SFP calling. SFP resequencing of 360 additional
accessions will follow in a third stage using duplicate arrays. With a total of 384 accessions a highresolution LD map will be obtained that traces back the fine ancestral recombination events within A.
thaliana. Even areas with reduced or no LD (<10kb seen at RPS5 (TIAN et al. 2002)), should be distinguished. For example ~30 SFPs may be found from over 500 covering a 10kb region. Thus, in contrast to SNP typing or sequencing at fixed distances, small haplotypes can be identified (see again Figure1). Population Genetic Analysis The SFP resequencing of 384 accessions will reveal empirical distributions for several population genetic parameters. We recognize that using proposed sampling strategy will result in a highly ascertained collection making it difficult or impossible to apply standard population genetic models. However rare fine patterns of variation seen in a genome scan can be further studied in new population samples. We note that empirical distributions from samples obtained so far clearly do not fit standard population models (NORDBORG et al. 2005; SCHMID et al. 2005) making it difficult to interpret results out of the whole genome context. θ, π, Tajima’s D, and the population genetic measure of recombination ρ, will be calculated from very high density SFP data providing an estimate for every gene and intergenic region. Unique patterns suggestive of selection can be chosen for further functional studies. Genomic analysis can give clues as to divergent patterns of variation seen among gene families (see preliminary data on R genes) and to chromosome regions. The relationship between population genetic statistics and measures of sequence repetition, genetic recombination, gene expression levels or variance could reveal potential evolutionary constraints. Linkage Disequilibrium and Haplotype analysis After SFP resequencing, LD patterns will be analyzed and haplotype blocks identified. Several approaches will be taken, including direct observation of pair-wise association patterns. One approach is simply to observe the pair-wise rate decay of LD with distance (DAWSON et al. 2002). This will be different for different regions of the chromosome, depending on recombination, demographic factors, and natural selection. Haplotype “block” calling algorithms can be used (DAWSON et al. 2002; GABRIEL et al. 2002; PATIL et al. 2001; ZHANG et al. 2002) to determine if the Arabidopsis genome is also organized into blocks, as seen in humans. These methods will take advantage of the very dense polymorphism data obtained from SFP resequencing. Other studies miss small haplotype blocks at the size of the inter-marker spacing (WALL and PRITCHARD 2003)(Figure 1). The population genetic recombination parameter ρ (HUDSON 1987) will be calculated along sliding windows using new multipoint approaches suitable for chromosome size data sets (LI and STEPHENS 2003) or methods tested for use with SFPs (JIANG et al. 2005). This will reveal recombination rate variation such as hot or cold spots in the genome and determine an empirical distribution for ρ. Selection will also influence the patterns on linkage disequilibrium and estimates of ρ. Recombination will be modeled after the Holliday Junction and gene conversion will also be accounted for (ANDOLFATTO and NORDBORG 1998; HAUBOLD et al. 2002). 18 RO1 GM073822 Principal Investigator/ Program Director: Borevitz, Justin Global Variation Reverse Genetic Resource We expect to identify more than 500,000 common SFPs (>1%), creating a comprehensive database
of saturating variation within the species. This will be made available on the web, (15X the current
density and 20X the sample size /sfp ). Molecular biologists studying
particular genes (via reverse genetics) could identify accessions containing SFPs and confirm the
specific changes by sequencing. By performing the appropriate crosses and scoring segregating
progeny, functional differences at candidate loci could be determined. Indeed as we have shown
hybridization variation can suggest candidate genes for QTL identified via association mapping or
traditional linkage mapping (WERNER et al. 2005)
Mitochondria and Chloroplast variation
Another important study will be to look at variation in maternally inherited chloroplast and
mitochondria genomes. Plants, and Arabidopsis in particular, have relatively large plastid genomes
(367kb mitochondria, and 154kb chloroplast) compared to mammals (rat, mouse, human 16kb). The
AtTILE1 array has approximately 25,000 features to interrogate all unique plastid sequences, a
fantastic tool to discover variation where little is expected. Importantly the plastid genomes do not
recombine. Here the phylogenetic signal has not been shuffled. This long stretch of sequence could
infer A. thaliana ancestry to position and date the common ancestor. In humans the low variation in
the small 16kb mitochondrial sequence traces “mitochondrial Eve” to ~60-80,000 years ago in Africa
(WATSON et al. 1997). The effects of seeds vs pollen dispersal can be investigated by comparing
mitochondria and chloroplast demographic patterns with nuclear genome-wide patterns. In addition,
association mapping can test cytoplasm genotype for effects on quantitative traits.
Improvements in Statistical methods of SFP genotyping for evolutionary analysis
The development of such a large data set, and its landmark use for evolutionary analysis and
association studies, warrants further research in optimization of SFP calling methods. Initial
improvements to SFP genotyping can be made using empirical Bayesian models such as those used
in the limma package of bioconductor for gene expression (SMYTH 2004). Here the data is first used
to estimate the total amount of SFPs, rather than assuming all the variance is due to noise. SFPs are
then identified under this prior probability model. This less restrictive null hypothesis assumes some
signal and some noise, while the current null hypothesis assumes that the data represents only noise.
Another level of improvement will be made with models that reflect what we know about
sequence variation. Here potential insertion/deletion polymorphisms (indels) are treated differently
from multiple single feature polymorphisms. This will reduce the current upward bias in the levels of
variation due to indels; they should not be counted as several independent SFPs when they really
represent single events. Deletions can be accounted for by considering the position of the 25 mer
feature in the genome when calling SFPs. An appropriate Hidden Markov Model (HMM) will consider
the state of the previous feature (defined as ‘same’ or ‘different’ from the reference genome) when
considering the genotype of the current feature. The extent to which prior information influences the
current test can depend on the distance between the features. It may be possible to recognize
several alleles of a single SFP with different intensities or occurring on different haplotype
backgrounds. The improvements made by these methods can be extensively verified as sequence
information is (http://walnut.usc.edu) (NORDBORG et al. 2005). Cross validation can be performed on
subsets of the sequence data and then be evaluated on the remaining subset.
Another improvement results from directly considering linkage disequilibrium among
polymorphisms when calling SFPs. Methods developed with this model look directly at the
hybridization intensity signature in a chromosome region. The pattern for a given region is then
compared to other strains (given the variation within replicates). In this way haplotypes are directly
called. This single step approach to genotyping and the determination of haplotype sharing may
reach the ultimate goal, which is to distinguish different chromosome types for use in association
19
RO1 GM073822 Principal Investigator/ Program Director: Borevitz, Justin
mapping. Successful implementation of this model will make SFP resequencing the preferred
approach to association studies in other sequenced organisms. SFP calling methods will be
incorporated into bioconductor packages such that they can take advantage of, and be integrated
into, other microarray analysis procedures. Further theoretical work on the properties of SFPs for
population genetic analysis will be performed as SFP calling methods are developed to make sure
they accurately measure nucleotide based statistics (JIANG et al. 2005).
Aim2 Genome wide association mapping using seasonal and geographic data
The SFP resequencing will be completed over the first two years. In addition to revealing fine
structure haplotypes and defining ancestral recombination breakpoints (discussed below), an inbred,
largely unstratified sample of this size should provide reasonable power for mapping adaptive traits
with high heritability (~80% broad sense), such as light response and flowering time. The extent of LD
is highly variable, but SFP resequencing data should identify even short range common haplotype
blocks (<5-10kb) (NORDBORG et al. 2002; NORDBORG et al. 2005; TIAN et al. 2002). Once identified, the localization of putative associations is limited by ancestral recombination events, as well as the frequency and magnitude of the effect. Here, new methods of LD mapping should make it possible to resolve large effect genes to a narrow region (HAGENBLAD et al. 2004; ZOLLNER and PRITCHARD 2005), resulting in a short list of candidate genes that can quickly be verified. We can take advantage of QTL mapping data for confirmation of genome wide associations, as many commonly used accessions will be included in our core set (http://www.inra.fr/qtlat/NaturalVar/RILSummary.htm). Association mapping can also be used for fine mapping of QTL identified using standard linkage mapping. An important consideration is that world-wide collections may have a high degree of genetic heterogeneity, such that several different genes (perhaps at low frequencies) all control variation. An additional problem is allelic heterogeneity, where several different alleles (possible rare) of the same gene have functional differences. In this case, clear phenotypic contrasts between haplotypes may not be obvious, although advanced algorithms may be able to account for this (HAGENBLAD et al. 2004; MOLITOR et al. 2003; ZOLLNER and PRITCHARD 2005). Most traits show broad variation across the native range which suggest that genetics determinant are also broadly distributed. As discussed above, a core set of generally equidistantly related accessions will be chosen to maximize the power and resolution for association mapping. Given the high density resequencing data, fine population structure will likely be revealed. This can then be accounted by using STRUCTURE (FEARNHEAD 2003). The final collection is likely to contain several of the commonly used world-wide accessions that have been previously studied. Data is available from large surveys of flowering time under several environments (STINCHCOMBE et al. 2004; WERNER et al. 2005), seedling light response (BOTTO and SMITH 2002; MALOOF et al. 2001), as well as seed and fruit quality traits (BENTSINK et al. 2000; BENTSINK et al. 2003). In addition we will collect data from unique seasonal and geographic environments from this complete set for light response and flowering time (below). Once a haplotype map is constructed for Arabidopsis thaliana, whole-genome association mapping can be performed. We have data to suggest that association mapping will be successful in Arabidopsis. Markers at the FRI and FLC loci show signs of being associated with flowering time (HAGENBLAD et al. 2004; STINCHCOMBE et al. 2004; WERNER et al. 2005) and will serve as positive controls for genome-wide scans. In addition markers at the major QTL, LIGHT1 and LIGHT2, (BOREVITZ et al. 2002) may be associated with light response across Arabidopsis accessions. The high density haplotype map and precise phenotype data from this model organism will be a testing ground for new LD mapping methods. Association mapping will be performed via genome-wide scans, testing linkage of the quantitative phenotype to individual haplotype blocks. As stated above, haplotype blocks, including small blocks that are important for fine mapping, will be well defined from SFP resequencing. This will give more power for association mapping. Very large sample sizes (1000s of individuals) may not be required to detect modest effect loci when polymorphisms are 20 RO1 GM073822 Principal Investigator/ Program Director: Borevitz, Justin comprehensively surveyed. This follows since to detect an association at a SNP that is not observed the sample size should be increased by 1/r2; r2 being the correlation between the observed and unobserved SNP. In addition, with inbred lines phenotypes can be replicated and traits are accurately measured in growth chambers. This is not considered for power studies in humans that suggest many thousands of individuals are required (WANG et al. 2005). Seasonal and Geographic light and flowering response The collection of 384 accessions will be made of up a majority of world-wide collections and some population-specific collections. They will be measured for quantitative variation in two well studied traits, seedling elongation light response and flowering time (FANKHAUSER and STAIGER 2002; KOMEDA 2004). The environments under which these traits will be studied will represent two seasons and two geographic locations. Winter annual accessions of A. thaliana germinate in the fall and over-winter as a rosette, then transition to flowering in the spring. Summer annual accessions in contrast germinate in the spring and more quickly set seeds in the summer. At southern latitudes, such as the possible glacial refugia in the Iberian Peninsula (SHARBEL et al. 2000), summer annual accessions may undergo two generations per year. In Northern latitudes likely only a single cycle is permitted. To capture the seasonal and geographic environmental variation under which A. thaliana has adapted, growth chamber settings will reflect light quality (spectral color composition), light intensity, day length, and temperature. Daily chamber settings are programmed to vary according to the changing season; either entering winter followed by spring for the fall planting, or spring conditions entering summer for the spring planting. Temperature, light quality and light intensity will cycle appropriately with changing day length regimes. Northern latitudes will be more extreme for seasonal variation while southern latitudes transition more gradually. The two seasonal planting times correspond to fall and spring, and the two geographic regions represent Spain and Sweden. These combinations create four environments that capture a variety of exogenous cues to signal flowering and seedling elongation (KOORNNEEF et al. 2004; MALOOF et al. 2000). Importantly, these settings capture the major environmental conditions in the field without the random noise that accompanies actual field conditions. These predictable seasonal changes are likely what plants can quickly adapt to, as compared to the more unpredictable disease and pest pressure, rainfall, or frost. Removing the unpredictable components in the environment reduces much of the noise in the experiment allowing one to focus on the genetic basis of adaptation to seasonal and geographic cues. 21 Day Length 0:00 2:00 4:00 6:00 8:00 10:00 12:00 14:00 16:00 18:00 20:00 22:00 sep oct nov dec jan feb mar apr may jun jul aug month hours Sw eden Spain standard standard Light Intensity 0 200 400 600 800 1000 1200 1400 sep oct nov dec jan feb mar apr may jun jul aug month W/m2 Sw eden Spain standard Temperature -10 -5 0 5 10 15 20 25 30 35 sep oct nov dec jan feb mar apr may jun jul aug month degrees C Spain High Spain Low Sw eden High Sw eden Low standard Figure 12 Seasonal and Geographic environments. Day length, light intensity (Hhttp://hurricane.ncdc.noaa.gov/CDO/cdoH) and temperature (http://dbsgap.versailles.inra.fr/vnat/) across difference geographic regions of the native Arabidopsis thaliana range. Growth chambers will be set to reflect the seasonal and geographic conditions. RO1 GM073822 Principal Investigator/ Program Director: Borevitz, Justin Molecular biologists may feel these environments are too complex and that it would be difficult to dissect the genetic basis of variation under these conditions. That may be true, but I would argue that studying flowering time under constant day length, light quantity, and light quality, and temperature does not make ecological sense (Figure 12). These are far from the conditions under which flowing time and seedling elongation responses have been selected upon. Thus it may be more appropriate to look at natural variation under conditions similar to field conditions. In this case the loci identified are much more likely to be ecologically relevant. Natural selection at these loci may have shaped patterns of variation. Thus, starting from environments that mimic wild conditions and perturbing them by season and geographic location may reveal a previously hidden genetic basis for differences in life history, such as winter annual vs. summer annual, and/or differences along latitudinal gradients that accurately reflect the genetic basis for adaptation in the field. The experimental design for this phenotypic display allows replicate measures of each of 384 accessions and an additional 30 known mutations. A single growth room will be set to a particular environment and each growth room can comfortably hold 5000 plants in individual pots. Each side of a single growth room will represent a single block replicate and within each block 6 individuals per genotype will be grown in a completely randomized design. Flats of 48 pots will be rotated across shelves and pots will be rotated within flats. Two growth rooms will be run in parallel, simulating first a spring planting in Northern and Southern latitudes, followed by the fall planting. The entire experiment will be repeated, switching Northern and Southern chambers and using new seed lots to control of general experimental variation and maternal effects. Each block will represent seed coming from separate mother plants. In total 6 plants per genotype X 414 genotypes X 2 blocks X 2 seasons X 2 geographic regions X 2 experiments =~ 40,000 plants. Flowering time will be measured in days until flowering, which is defined as the date at which the primary stem is 1cm above the rosette. This measure is highly correlated with total leaf number at flowering, another commonly used measure of developmental age. The heritability of flowering time has been shown to be greater the 90% (ALONSOBLANCO et al. 1998). The growth chambers are now running smoothly, and we have implemented automated watering schedules and humidity control to further mimic seasonal averages. A wireless barcode reader is being used to quickly and anonymously capture the flowering time of each pot on a daily basis. This robust phenotypic data set will be the platform upon which association mapping studies will be performed. It will also reveal many interesting accessions for future genetic studies. The phenotypic data analysis will be performed using general linear models, fitting main effects of genotype, season, geographic location. The interaction terms will reveal how specific genotypes behave differently across seasons and locations or how a genotype response depends on both season and location. This analysis allows the specific environmental responses to be decomposed. The genetic basis of the environmental response, rather than absolute flowering time or seedling light response, may be less complex, revealing specific loci controlling adaptation to different environments. These additional traits could be used for association mapping. Alternatively multi-trait mapping approaches could be developed such as those used in QTL mapping (JIANG and ZENG 1995). The second trait of interest that will be studied is seedling length light response. We and others have found substantial genetic variation among A. thaliana accessions and unique patterns of variation across light conditions (BOTTO and SMITH 2002; MALOOF et al. 2001). This trait may be important for fitness relating to seedling emergence or density dependant growth (MALOOF et al. 2000) and is quickly measured in the lab. Heritability for seedling length is also high (>70%) across
accessions (MALOOF et al. 2001) or in segregation populations (BOREVITZ et al. 2002). I propose to
also measure seedling length light response in the same seasonal and geographic environments as
for flowering time. This represents day lengths found at the time of germination, light quality and light
quantity that change during the course of the day in a manner quite close to an actual fall or spring
days. The flowering time and light response experiments will be coordinated so that after 10 days
22
RO1 GM073822 Principal Investigator/ Program Director: Borevitz, Justin
some seedlings will be transferred from plates to soil for flowering measurements and the length of
~20 seedlings will be directly measured for light response. We also have benchtop growth chambers
with identical lighting, temperature, and humidity controls to those in the walk in rooms, so that
seedling experiments can be replicated more often or under a wider array of seasonal cues.
Core resource for association mapping
The high resolution haplotype map developed under Aim1 is the ultimate genetic resource. It is an
immortal mapping population upon which many traits can be analyzed by the entire Arabidopsis
community. Seeds are easily saved and distributed from stock centers. In addition the community
developing methods for association mapping can test new approaches with hopes to identify novel
smaller effect loci and interactions between loci while accounting for known large effect loci. It is
likely that the most effective methods of linkage disequilibrium mapping will test for significant
associations between haplotype blocks and the quantitative phenotype rather than single multi
marker analysis. Importantly, in Arabidopsis, particular associations can be experimentally
verified (Aim3) to discover new genes and new alleles of known genes.
Association mapping will identify regions of the genome important for variation in quantitative
traits. We will perform association mapping using the latest algorithms for our complete survey of
light response and flowering time data across different environments. We will also map the
environmental and geographic responses that can be dissected from the analysis of variance across
environments. Finally additional quantitative traits from other groups will also be mapped. This
includes our original survey of light response under specific light quality conditions (MALOOF et al.
2001), flowering time under particular constant conditions of day length (WERNER et al. 2005) ambient
temperature (Janne Lempe and Detlef Weigel, submitted to PLoS), vernalization response (Magnus
Nordborg, and Caroline Dean, unpublished), survivorship and flowering time under field conditions
(STINCHCOMBE et al. 2004) and other traits/environments conducted by other groups.
Using SFPs for association mapping
The main advantage of SFP re-sequencing on tiling arrays for association mapping is that it makes it
possible to achieve a very high marker density. Based on current data, we predict that will have a
common SFP (> 5%) every 300 bp throughout the genome. In an organism in which LD decays over
25-50 kb on average (Nordborg et al, 2005), our power to pick up genotype-phenotype associations
due to LD will be very high. SNP-based assays could never achieve this marker density, and would
consequently risk “missing” associations. In addition, non SNP-based variants, such as small
deletions that disrupt potential coding or regulatory regions, will also be surveyed. These are, by
definition, missed by SNP methods.
At the same time, it is important to realize that the data we propose to generate will be far from
complete re-sequencing data. Preliminary studies have indicated that although false positives occur
at the specified rate (i.e. called SFPs are reliable), false negatives are frequent (the fact that an
individual has an allele different from the reference genome is not always detected). From the point
of view of association mapping, this is very important. If we had perfect data, LD becomes irrelevant:
we would simply search for associations between all polymorphisms (which would include the
functionally important ones) and the phenotype. The importance of LD lies in the fact that it provides
power to infer the location of functionally important loci we have not observed.
For SFP data we are faced with the challenging problem that the functionally important site or
sites may or may not be included in the data. Furthermore, even if such a site is included, a fraction
of individuals will be misclassified. Currently, multiple alleles also cannot be distinguished, such as
multiple closely linked SNPs, or different indel polymorphisms, although this occurs less than 18% of
the time. Fortunately, high marker density and LD comes to the rescue. Because we will have a very
large number of SFPs in LD with any particular functionally important polymorphism, we should
normally be able to infer the underlying haplotype structure and overcome the problem of missing or
23
RO1 GM073822 Principal Investigator/ Program Director: Borevitz, Justin
misclassified data. This basic problem is the same as for SNP data that is more accurate but much
less dense. In both cases we search for haplotypes associated with phenotypes: with SNP data, the
challenge is to use LD to compensate for the fact that we only observe a small fraction of all
polymorphisms; with SFP data, the challenge is using LD to compensate for the fact that, although we
observe most polymorphisms, we observe them imperfectly.
We propose to accomplish this by using algorithms similar to those we have used successfully
when mapping Arabidopsis SNP data (HAGENBLAD et al. 2004). The performance of such algorithms
depends crucially upon the choice of similarity measure. When using SNPs our similarity metrics can
be relatively simple pairwise comparisons between SNPs. In the context of SFPs, it will be
appropriate to modify the similarity metric in such a way that it is able to infer similarity between SFP
‘haplotypes’ even in the presence of false-negatives. Without LD this would be very challenging, but
the presence of extensive LD will allow such inference when information from several nearby SFPs is
combined. We will explore a range of different similarity metrics in order to find one with good power
in this context. Since theoretical results for the behavior of such algorithms, and their associated
metrics, are likely to prove very challenging to derive, performance of metrics, and null-distributions of
test statistics will be assessed via repeated analysis of resampled data sets. Given the inherent
randomness introduced to the data by false-negatives, we will also explore extensions of our methods
in which clustering occurs in a probabilistic rather than deterministic fashion. This will also allow the
exploration of haplotype clusterings that, despite appearing superficially less likely because of
differences introduced by false-positives, in fact represent true underlying similarities between the
unobserved nucleotide sequences. Since SFPs are quantitative the effect of different error rates can
then be studied.
AIM3 Identify the genetic basis underlying associations from the genome scan.
Under Aim3 we will determine the genetic basis for major variation in flowering time and light
response, taking advantage of powerful genetic resources available in Arabidopsis (BOREVITZ 2005;
BOREVITZ et al. 2003). Particular focus will be on loci that show interaction with the seasonal and/or
geographic environments. Both traits will be investigated; here I describe the approach using the
flowering time trait. Associations will reveal loci and their interaction with the environment. The
likelihood of the association and size of the region detected will depend on the magnitude of the
genetic effect, the allele frequency across the 384 accessions, and the amount of linkage
disequilibrium in the region (i.e. the amount of ancestral recombination). The approach used to
verify the association and confirm the precise genetic cause will depend on the confidence
interval at the QTL. Regions could be as large as 1Mb for a rare small effect locus with little
recombination or as small as 10kb for a common large effect locus that has undergone extensive
ancestral recombination. In the later case the approach is to directly investigate candidate genes
(see below). For intervals that span greater than 10 genes (>50kb), our experience suggests that,
given the amount of work to confirm candidate genes, these should first be confirmed and fine
mapped in segregating populations. Although it is temping to select only large effect loci for
confirmation we will pursue at least one small effect putative association (<10% variance explained)
as these are likely to make up the majority of regions detected in a genome scan for complex traits.
However, when large effect loci are confirmed their variance can be controlled for in future genome
scans, providing more power to reveal small effect loci (ZENG 1994). We stress again that the goal of
this proposal is to verify whole genome association mapping results and develop the approach as a
viable one in Arabidopsis, as an example for other organisms. Thus, the approaches below could be
used without association mapping to get to the genes, which is exactly the point.
Confirmation of association in segregating cross.
In some cases QTL identified from a genome-wide association scan will co-localize with QTL
identified through mapping in a particular cross (LIGHT1 and LIGHT2 see preliminary result and FRI
24
RO1 GM073822 Principal Investigator/ Program Director: Borevitz, Justin
and FLC (HAGENBLAD et al. 2004)). Particular attention should be paid to determining which
haplotypes are functionally different in the association scan. This should match with the parental
lines used for traditional QTL mapping. When this occurs, it is further evidence that the association is
real. Then the particular mapping cross can be used or we can perform further fine mapping as
necessary.
Novel loci identified in genome-wide association scans will be confirmed by choosing parental
lines that differ in predicted functional genotypes at the QTL. Three different crosses will be made to
verify linkage at the locus to try and account for possible background effects. 500 plants in the F2
generation will be screened for the quantitative phenotype, after which extreme plants will be pooled
and genotyped in bulk using eXtreme Array Mapping (XAM) (WOLYN et al. 2004) (WERNER et al.
2005). When each cross identifies the same locus that was detected in the original LD scan, we
conclude that the association is real and proceed to the next stage. Recombination fine mapping will
be used to narrow the region to a small interval (< 50kb). This is trivial in Arabidopsis. In our
experience, this is the most direct and interpretable method to refine the genetic locus. To improve
the resolution of XAM, recombinant plants will be identified that span the QTL by PCR genotyping two
flanking markers. A minimum of 2000 plants will be screened for genotype and only progeny from the
recombinants will be phenotyped to determine the inherited QTL allele. At this stage the rate limiting
step is the determination of the precise recombination breakpoints in each recombinant. Here the
high density SFP genotypes will be exploited in a second round of XAM. Once non-recombinant
chromosomes are removed from pools of lines with either QTL allele, XAM will have the precision to
reveal fine scale recombination events due to the abundance of SFP markers in a given region
(<1kb). This two stage XAM strategy will be extensively implemented under this proposal.
Candidate gene approach
Once a narrow QTL interval is well defined, candidate genes will be identified. Several lines of
evidence will be used to suggest a gene and/or causative polymorphism, but the final confirmation will
require transgenic complementation (BOREVITZ and CHORY 2004).
1) Transcriptional variation will be determined by profiling lines with different QTL alleles, testing
different environments, tissues, and/or developmental stages using AtTILE1. This will both
characterize the downstream transcriptional response of the QTL (differentially expressed
genes unlinked to the QTL) and suggest candidate genes as differentially expressed genes
mapping to the QTL. One can use near isogenic lines, heterogenous inbred families (LOUDET
et al. 2002) or pools on extreme recombinant lines to compare transcriptional variation
between genotypes and across environments. Importantly, gene expression variation will
NOT be confounded by hybridization variation as this will be accounted for during SFP
resequencing. In addition we will screen a publicly available gene expression atlas to
determine the expression profile of candidate genes. Currently, this includes over 1400 high
quality affymetrix gene expression arrays (http://affymetrix.arabidopsis.info/ ,
http://arabidopsis.org), representing a developmental and stress time courses, and both new
and old circadian rhythm studies (Sam Hazen, personal communication) (HARMER et al. 2000).
The published tiling array data set from four tissues can also be investigated at the candidate
locus for novel transcription, alternative splicing, and antisense transcriptions,
http://blast1.salk.edu/cgi-bin/AtGE (YAMADA et al. 2003), Lastly, the Massively Parallel
Signature Sequences can be screened http://mpss.udel.edu/at/ for interesting patterns of
transcriptional variation.
2) The QTL interval will be screened for insertion/deletion and SFPs polymorphisms that may
disrupt or alter candidate gene function (WERNER et al. 2005). The SFP resequencing data
from AtTILE1 (Aim1, haplotype map) will reveal near saturating polymorphism across the
candidate region. Patterns of variation that suggest balancing or diversifying selection can
also be used to select a specific candidate gene that may have been shaped by evolution.
25
RO1 GM073822 Principal Investigator/ Program Director: Borevitz, Justin
3) Molecular genetic knowledge of flowering time and light response signal transduction pathway
can suggest candidate genes in Arabidopsis (FANKHAUSER and STAIGER 2002; KOMEDA 2004).
This point should not be taken for granted, as these molecular signal transduction pathways
have been extensively characterized in plants.
4) Screening of knockout lines of all genes in the interval (ALONSO et al. 2003; SESSIONS et al.
2002) for quantitative phenotypes can suggest candidate genes (http://signal.salk.edu).
Currently over 342,000 sequenced indexed insertion lines are available in multiple genetic
backgrounds, with most genes represented by more than 1 allele. A null mutant background
can be used for quantitative complementation tests with natural alleles and for transgenic
experiments.
5) Finally, direct sequencing and transgenic complementation will be used to confirm that the
QTL has been cloned. Early stop codons or changes in a potential active site suggest
functional consequences, as do changes that are not evolutionarily conserved when compared
with orthologues of other plants (EL-DIN EL-ASSAL et al. 2001; MALOOF et al. 2001). To
determine the functional differences between various natural alleles, they will be transformed
into a null background using genomic constructs. Hundreds of primary transgenic lines will be
screened for quantitative phenotypes to average out the position effect. Alternatively, multiple
transgenic lines can be progeny tested. Identifying the molecular basis of a complex trait will
improve our knowledge of light or flowering signal transduction and illustrate a successful path
toward genom-wide LD mapping. The lessons learned will be invaluable to other LD studies
that attempt to identify genes underlying complex disease
6) Once a gene responsible for a QTL has been identified, we will determine which polymorphism
or combination of polymorphisms is responsible for the QTL. This will require construction of
novel alleles which can then be reintroduced transgenically. If epistatic interactions with the
QTL and background are identified, transgenic experiments can be performed in both
backgrounds.
TIMELINE
Year 1 – Aim 1 – All available accessions will be screened to identify siblings with the Ohio State stock center.
A non-redundant set will be genotyped at 384 moderate frequency high Fst SNPs to determine gross
population structure. The final choice of 384 largely unstructured core accessions will be identified from 2
populations. Preliminary SFP resequencing will be performed with additional replication on reference and a
minimum of 22 lines to determine suitable power and call common alleles. We will begin SFP resequencing of
the remaining lines. Improved SFP calling methods, including simulation studies to determine thier effect on
population genetic statistics will be performed. Aim2 Phenotypic studies of the core set will begin under
simulated “spring” conditions in both the “Northern” and “Southern” geographic location.
Year 2 – Aim 1 – Complete SFP resequencing of 384 accessions. Analyze patterns of variation, ancestral
recombination events, determine fine genome-wide distributions for population genetic statistics and scan for
loci potentially under selection. Aim2 – Perform first genome-wide LD scans with publicly available phenotypic
data and the first replicate of seasonal data from year1. Refine methods using known loci as positive controls.
Perform “fall” seasonal phenotypic screen of core collection, and 2nd replicate of “spring” conditions in both
geographic environments.
Year 3 – Aim 2 – Complete final replicate of “fall” planting. Perform advanced methods of genome wide
association mapping using full seasonal and geographic data sets. Aim3 choose putative loci from genome
wide scan for follow up studies, analyze several crosses using eXtreme Array Mapping, and fine recombination
array mapping where needed. Look at pools of extreme lines or isogenic lines for variation in gene expression
to suggest candidate loci. Control for hybridization variation in gene expression between genotypes using
SFP resequencing data.
Year 4 – Aim 3 – Fine map and clone genes involved in light response or natural flowering time via direct
sequencing, quantitative complementation and analysis of knock out lines. Use this information to further
improve genome wide association scans.
Year 5 – Aim 3 – Characterize candidate genes for functional variation in flowering time or light response, via
gene expression and alternative splicing studies.
26
RO1 GM073822 Principal Investigator/ Program Director: Borevitz, Justin
e. Human Subjects
NONE
f. Vertebrate Animals
NONE
g. Literature Cited
AKEY, J. M., K. ZHANG, M. XIONG and L. JIN, 2003 The effect of single nucleotide polymorphism identification
strategies on estimates of linkage disequilibrium. Mol Biol Evol 20: 232-242.
ALONSO-BLANCO, C., S. E.-D. EL-ASSAL, G. COUPLAND and M. KOORNNEEF, 1998 Analysis of natural allelic
variation at flowering time loci in the Landsberg erecta and Cape Verde Islands ecotypes of Arabidopsis
thaliana. Genetics 149: 749-764.
ALONSO, J. M., A. N. STEPANOVA, T. J. LEISSE, C. J. KIM, H. CHEN et al., 2003 Genome-wide insertional
mutagenesis of Arabidopsis thaliana. Science 301: 653-657.
ANDOLFATTO, P., and M. NORDBORG, 1998 The effect of gene conversion on intralocus associations. Genetics
148: 1397-1399.
BENTSINK, L., C. ALONSO-BLANCO, D. VREUGDENHIL, K. TESNIER, S. P. GROOT et al., 2000 Genetic analysis of
seed-soluble oligosaccharides in relation to seed storability of Arabidopsis. Plant Physiol 124: 1595-
1604.
BENTSINK, L., K. YUAN, M. KOORNNEEF and D. VREUGDENHIL, 2003 The genetics of phytate and phosphate
accumulation in seeds and leaves of Arabidopsis thaliana, using natural variation. Theor Appl Genet
106: 1234-1243.
BERGELSON, J., M. KREITMAN, E. A. STAHL and D. TIAN, 2001 Evolutionary dynamics of plant R-genes.
Science 292: 2281-2285.
BOLSTAD, B. M., R. A. IRIZARRY, M. ASTRAND and T. P. SPEED, 2003 A comparison of normalization methods
for high density oligonucleotide array data based on variance and bias. Bioinformatics 19: 185-193.
BOREVITZ, J. O., 2005 Array Genotyping and Mapping. Arabidopsis Protocols (in press):
/methods. BOREVITZ, J. O., and J. CHORY, 2004 Genomics tools for QTL analysis and gene discovery. Curr Opin Plant
Biol 7: 132-136.
BOREVITZ, J. O., and J. R. ECKER, 2004 Plant genomics: the third wave. Annu Rev Genomics Hum Genet 5:
443-477.
BOREVITZ, J. O., D. LIANG, D. PLOUFFE, H. S. CHANG, T. ZHU et al., 2003 Large-scale identification of singlefeature polymorphisms in complex genomes. Genome Res 13: 513-523.
BOREVITZ, J. O., J. N. MALOOF, J. LUTES, T. DABI, J. L. REDFERN et al., 2002 Quantitative Trait Loci
Controlling Light and Hormone Response in Two Accessions of Arabidopsis thaliana. Genetics 160:
683-696.
BOREVITZ, J. O., and M. NORDBORG, 2003 The impact of genomics on the study of natural variation in
Arabidopsis. Plant Physiology 132: 718-725.
BOTTO, J. F., and H. G. SMITH, 2002 Differential genetic variation in adaptive strategies to a common
environmental signal in Arabidopsis accessions; phytochrome-mediated shade avoidance. Plant Cell and
Environment 25: 53-63.
DAWSON, E., G. R. ABECASIS, S. BUMPSTEAD, Y. CHEN, S. HUNT et al., 2002 A first-generation linkage
disequilibrium map of human chromosome 22. Nature.
EL-DIN EL-ASSAL, S., C. ALONSO-BLANCO, A. J. PEETERS, V. RAZ and M. KOORNNEEF, 2001 A QTL for
flowering time in Arabidopsis reveals a novel allele of CRY2. Nat Genet 29: 435-440.
FALUSH, D., M. STEPHENS and J. K. PRITCHARD, 2003 Inference of population structure using multilocus
genotype data: linked loci and correlated allele frequencies. Genetics 164: 1567-1587.
FANKHAUSER, C., and D. STAIGER, 2002 Photoreceptors in Arabidopsis thaliana: light perception, signal
transduction and entrainment of the endogenous clock. Planta 216: 1-16.
FEARNHEAD, P., 2003 Consistency of estimators of the population-scaled recombination rate. Theor Popul Biol
64: 67-79.
FLINT-GARCIA, S. A., J. M. THORNSBERRY and E. S. T. BUCKLER, 2003 Structure of linkage disequilibrium in
plants. Annu Rev Plant Biol 54: 357-374.
27
RO1 GM073822 Principal Investigator/ Program Director: Borevitz, Justin
FRANSZ, P. F., S. ARMSTRONG, J. H. DE JONG, L. D. PARNELL, C. VAN DRUNEN et al., 2000 Integrated
cytogenetic map of chromosome arm 4S of A. thaliana: structural organization of heterochromatic knob
and centromere region. Cell 100: 367-376.
FREEDMAN, M. L., D. REICH, K. L. PENNEY, G. J. MCDONALD, A. A. MIGNAULT et al., 2004 Assessing the
impact of population stratification on genetic association studies. Nat Genet 36: 388-393.
GABRIEL, S. B., S. F. SCHAFFNER, H. NGUYEN, J. M. MOORE, J. ROY et al., 2002 The structure of haplotype
blocks in the human genome. Science 296: 2225-2229.
GOLDSTEIN, D. B., and M. E. WEALE, 2001 Population genomics: linkage disequilibrium holds the key. Curr
Biol 11: R576-579.
HAGENBLAD, J., C. TANG, J. MOLITOR, J. WERNER, K. ZHAO et al., 2004 Haplotype structure and phenotypic
associations in the chromosomal regions surrounding two Arabidopsis thaliana flowering time loci.
Genetics 168: 1627-1638.
HARMER, S. L., J. B. HOGENESCH, M. STRAUME, H. S. CHANG, B. HAN et al., 2000 Orchestrated transcription of
key pathways in Arabidopsis by the circadian clock. Science 290: 2110-2113.
HAUBOLD, B., J. KROYMANN, A. RATZKA, T. MITCHELL-OLDS and T. WIEHE, 2002 Recombination and gene
conversion in a 170-kb genomic region of Arabidopsis thaliana. Genetics 161: 1269-1278.
HAZEN, S. P., J. O. BOREVITZ, F. G. HARMON, J. L. PRUNEDA-PAZ, T. F. SCHULTZ et al., 2005 Rapid array
mapping of circadian clock and developmental mutations in Arabidopsis. Plant Physiology (in press):
/methods.
HEKSTRA, D., A. R. TAUSSIG, M. MAGNASCO and F. NAEF, 2003 Absolute mRNA concentrations from
sequence-specific calibration of oligonucleotide arrays. Nucleic Acids Res 31: 1962-1968.
HINDS, D. A., A. B. SEYMOUR, L. K. DURHAM, P. BANERJEE, D. G. BALLINGER et al., 2004 Application of
pooled genotyping to scan candidate regions for association with HDL cholesterol levels. Hum
Genomics 1: 421-434.
HINDS, D. A., L. L. STUVE, G. B. NILSEN, E. HALPERIN, E. ESKIN et al., 2005 Whole-genome patterns of
common DNA variation in three human populations. Science 307: 1072-1079.
HUDSON, R. R., 1987 Estimating the recombination parameter of a finite population model without selection.
Genet Res 50: 245-250.
INTERNATIONAL_HAPMAP_PROJECT, 2003 The International HapMap Project. Nature 426: 789-796.
JIANG, C., and Z. B. ZENG, 1995 Multiple trait analysis of genetic mapping for quantitative trait loci. Genetics
140: 1111-1127.
JIANG, R., J. O. BOREVITZ, P. MARJORAM and S. TAVARE, 2005 Inferring Population History From SingleFeature Polymorphism Data. to be submitted to Genetics:
/haplotype/sfpTheory.pdf.
KOMEDA, Y., 2004 Genetic Regulation of Time to Flower in Arabidopsis thaliana. Annu Rev Plant Physiol
Plant Mol Biol 55: 521-535.
KOORNNEEF, M., C. ALONSO-BLANCO and D. VREUGDENHIL, 2004 Naturally Occurring Genetic Variation in
Arabidopsis Thaliana. Annu Rev Plant Physiol Plant Mol Biol 55: 141-172.
KRUGLYAK, L., 1999 Prospects for whole-genome linkage disequilibrium mapping of common disease genes.
Nat Genet 22: 139-144.
LI, N., and M. STEPHENS, 2003 Modeling linkage disequilibrium and identifying recombination hotspots using
single-nucleotide polymorphism data. Genetics 165: 2213-2233.
LONG, A. D., R. F. LYMAN, C. H. LANGLEY and T. F. MACKAY, 1998 Two sites in the Delta gene region
contribute to naturally occurring variation in bristle number in Drosophila melanogaster. Genetics 149:
999-1017.
LOUDET, O., S. CHAILLOU, C. CAMILLERI, D. BOUCHEZ and F. DANIEL-VEDELE, 2002 Bay-0 x Shahdara
recombinant inbred line population: a powerful tool for the genetic dissection of complex traits. Theor.
Appl. Genet. 104: 1173-1184.
MACKAY, T. F., 2001 The genetic architecture of quantitative traits. Annu Rev Genet 35: 303-339.
MALOOF, J. N., J. O. BOREVITZ, T. DABI, J. LUTES, R. B. NEHRING et al., 2001 Natural variation in light
sensitivity of Arabidopsis. Nat Genet 29: 441-446.
MALOOF, J. N., J. O. BOREVITZ, D. WEIGEL and J. CHORY, 2000 Natural variation in phytochrome signaling.
Semin Cell Dev Biol 11: 523-530.
MARCHINI, J., L. R. CARDON, M. S. PHILLIPS and P. DONNELLY, 2004 The effects of human population structure
on large genetic association studies. Nat Genet 36: 512-517.
MAURICIO, R., E. A. STAHL, T. KORVES, D. TIAN, M. KREITMAN et al., 2003 Natural selection for polymorphism
in the disease resistance gene Rps2 of Arabidopsis thaliana. Genetics 163: 735-746.
28
RO1 GM073822 Principal Investigator/ Program Director: Borevitz, Justin
MEYERS, B. C., A. KOZIK, A. GRIEGO, H. KUANG and R. W. MICHELMORE, 2003 Genome-wide analysis of
NBS-LRR-encoding genes in Arabidopsis. Plant Cell 15: 809-834.
MITRA, R. M., C. A. GLEASON, A. EDWARDS, J. HADFIELD, J. A. DOWNIE et al., 2004 A Ca2+/calmodulindependent protein kinase required for symbiotic nodule development: Gene identification by transcriptbased cloning. Proc Natl Acad Sci U S A 101: 4701-4705.
MOLITOR, J., P. MARJORAM and D. THOMAS, 2003 Fine-scale mapping of disease genes with multiple mutations
via spatial clustering techniques. Am J Hum Genet 73: 1368-1384.
NASRALLAH, M. E., P. LIU and J. B. NASRALLAH, 2002 Generation of self-incompatible Arabidopsis thaliana by
transfer of two S locus genes from A. lyrata. Science 297: 247-249.
NORDBORG, M., 2000 Linkage disequilibrium, gene trees and selfing: an ancestral recombination graph with
partial self-fertilization. Genetics 154: 923-929.
NORDBORG, M., J. O. BOREVITZ, J. BERGELSON, C. C. BERRY, J. CHORY et al., 2002 The extent of linkage
disequilibrium in Arabidopsis thaliana. Nat Genet 30: 190-193.
NORDBORG, M., T. HU, Y. ISHINO, J. JHAVERI, C. TOOMAJIAN et al., 2005 The Pattern of Polymorphism in
Arabidopsis thaliana. PLoS: http://walnut.usc.edu/~magnus/2010-PLoS.pdf.
PADHUKASAHASRAM, B., P. MARJORAM and M. NORDBORG, 2004 Estimating the rate of gene conversion on
human chromosome 21. Am J Hum Genet 75: 386-397.
PATIL, N., A. J. BERNO, D. A. HINDS, W. A. BARRETT, J. M. DOSHI et al., 2001 Blocks of limited haplotype
diversity revealed by high-resolution scanning of human chromosome 21. Science 294: 1719-1723.
PHILLIPS, M. S., R. LAWRENCE, R. SACHIDANANDAM, A. P. MORRIS, D. J. BALDING et al., 2003 Chromosomewide distribution of haplotype blocks and the role of recombination hot spots. Nat Genet 33: 382-387.
RATCLIFFE, O. J., G. C. NADZAN, T. L. REUBER and J. L. RIECHMANN, 2001 Regulation of flowering in
Arabidopsis by an FLC homologue. Plant Physiol 126: 122-132.
REICH, D. E., M. CARGILL, S. BOLK, J. IRELAND, P. C. SABETI et al., 2001 Linkage disequilibrium in the human
genome. Nature 411: 199-204.
RISCH, N., and K. MERIKANGAS, 1996 The future of genetic studies of complex human diseases. Science 273:
1516-1517.
RONALD, J., J. M. AKEY, J. WHITTLE, E. N. SMITH, G. YVERT et al., 2005 Simultaneous genotyping, geneexpression measurement, and detection of allele-specific expression with oligonucleotide arrays.
Genome Res 15: 284-291.
ROSTOKS, N., J. O. BOREVITZ, P. E. HEDLEY, J. RUSSELL, S. MUDIE et al., 2005 Single Feature Polymorphism
discovery in the barley transcriptome. (submitted to Genome Biology 2/7/05):
/barley.
SCHMID, K. J., S. RAMOS-ONSINS, H. RINGYS-BECKSTEIN, B. WEISSHAAR and T. MITCHELL-OLDS, 2005 A
multilocus sequence survey in Arabidopsis thaliana reveals a genome-wide departure from the standard
neutral model of DNA sequence polymorphism. Genetics.
SCORTECCI, K. C., S. D. MICHAELS and R. M. AMASINO, 2001 Identification of a MADS-box gene,
FLOWERING LOCUS M, that represses flowering. Plant J. 26: 229-236.
SESSIONS, A., E. BURKE, G. PRESTING, G. AUX, J. MCELVER et al., 2002 A high-throughput Arabidopsis reverse
genetics system. Plant Cell 14: 2985-2994.
SHARBEL, T. F., B. HAUBOLD and T. MITCHELL-OLDS, 2000 Genetic isolation by distance in Arabidopsis
thaliana: Biogeography and postglacial colonization of Europe. Molecular Ecology 9: 2109-2118.
SMYTH, G., 2004 Linear Models and Empirical Bayes Methods for Assessing Differential Expression in
Microarray Experiments. Statist Applications Genet Mol Biology 3.
STAHL, E. A., G. DWYER, R. MAURICIO, M. KREITMAN and J. BERGELSON, 1999 Dynamics of disease resistance
polymorphism at the Rpm1 locus of Arabidopsis. Nature 400: 667-671.
STINCHCOMBE, J. R., C. WEINIG, M. UNGERER, K. M. OLSEN, C. MAYS et al., 2004 A latitudinal cline in
flowering time in Arabidopsis thaliana modulated by the flowering time gene FRIGIDA. Proc Natl Acad
Sci U S A 101: 4712-4717.
SWARUP, K., C. ALONSO-BLANCO, J. R. LYNN, S. D. MICHAELS, R. M. AMASINO et al., 1999 Natural allelic
variation identifies new genes in the Arabidopsis circadian system. Plant Journal 20: 67-77.
TAJIMA, F., 1983 Evolutionary relationship of DNA sequences in finite populations. Genetics 105: 437-460.
TAJIMA, F., 1989 Statistical method for testing the neutral mutation hypothesis by DNA polymorphism.
Genetics 123: 585-595.
TAVARE, S., D. J. BALDING, R. C. GRIFFITHS and P. DONNELLY, 1997 Inferring coalescence times from DNA
sequence data. Genetics 145: 505-518.
THORNSBERRY, J. M., M. M. GOODMAN, J. DOEBLEY, S. KRESOVICH, D. NIELSEN et al., 2001 Dwarf8
29
RO1 GM073822 Principal Investigator/ Program Director: Borevitz, Justin
polymorphisms associate with variation in flowering time. Nat Genet 28: 286-289.
TIAN, D., M. B. TRAW, J. Q. CHEN, M. KREITMAN and J. BERGELSON, 2003 Fitness costs of R-gene-mediated
resistance in Arabidopsis thaliana. Nature 423: 74-77.
TIAN, D. C., H. ARAKI, E. STAHL, J. BERGELSON and M. KREITMAN, 2002 Signature of balancing selection in
Arabidopsis. Proceedings of the National Academy of Sciences of the United States of America 99:
11525-11530.
TUSHER, V. G., R. TIBSHIRANI and G. CHU, 2001 Significance analysis of microarrays applied to the ionizing
radiation response. Proc Natl Acad Sci U S A 98: 5116-5121.
WALL, J. D., and J. K. PRITCHARD, 2003 Haplotype blocks and linkage disequilibrium in the human genome.
Nat Rev Genet 4: 587-597.
WANG, W. Y., B. J. BARRATT, D. G. CLAYTON and J. A. TODD, 2005 Genome-wide association studies:
theoretical and practical concerns. Nat Rev Genet 6: 109-118.
WATSON, E., P. FORSTER, M. RICHARDS and H. J. BANDELT, 1997 Mitochondrial footprints of human
expansions in Africa. Am J Hum Genet 61: 691-704.
WATTERSON, G. A., 1975 On the number of segregating sites in genetical models without recombination. Theor.
Popul. Biol. 7: 256-276.
WERNER, J. D., J. O. BOREVITZ, H. N. UHLENHAUT, J. R. ECKER, J. CHORY et al., 2005 FRIGIDA-independent
variation in flowering time of natural A. thaliana accessions. Genetics (in press):
/werner.
WERNER, J. D., J. O. BOREVITZ, N. WARTHMANN, G. T. TRAINER, J. R. ECKER et al., 2005 Quantitative trait
locus mapping and DNA array hybridization identify an FLM deletion as a cause for natural floweringtime variation. Proc Natl Acad Sci U S A 102: 2460-2465.
WINZELER, E. A., D. R. RICHARDS, A. R. CONWAY, A. L. GOLDSTEIN, S. KALMAN et al., 1998 Direct allelic
variation scanning of the yeast genome. Science 281: 1194-1197.
WOLYN, D. J., J. O. BOREVITZ, O. LOUDET, C. SCHWARTZ, J. MALOOF et al., 2004 Light-response quantitative
trait loci identified with composite interval and eXtreme array mapping in Arabidopsis thaliana.
Genetics 167: 907-917.
WU, Z., and R. A. IRIZARRY, 2004 Stochastic Models Inspired by Hybridization Theory for Short
Oligonucleotide Arrays. Proceedings of RECOMB.
YAMADA, K., J. LIM, J. M. DALE, H. CHEN, P. SHINN et al., 2003 Empirical analysis of transcriptional activity in
the Arabidopsis genome. Science 302: 842-846.
ZENG, Z. B., 1994 Precision mapping of quantitative trait loci. Genetics 136: 1457-1468.
ZHANG, K., M. DENG, T. CHEN, M. S. WATERMAN and F. SUN, 2002 A dynamic programming algorithm for
haplotype block partitioning. Proc Natl Acad Sci U S A 99: 7335-7339.
ZHANG, L., M. F. MILES and K. D. ALDAPE, 2003 A model of molecular interactions on short oligonucleotide
microarrays. Nat Biotechnol 21: 818-821.
ZHANG, W., A. COLLINS and N. E. MORTON, 2004 Does haplotype diversity predict power for association
mapping of disease susceptibility? Hum Genet 115: 157-164.
ZOLLNER, S., and J. K. PRITCHARD, 2005 Coalescent-based association mapping and fine mapping of complex
trait loci. Genetics.
h. Consortium/Contractual Agreements
USC collaborator Paul Marjoram: Paul Marjoram has developed methods for association mapping in
Arabidopsis and developed theory for using SFPs in molecular population genetics. Paul will continue
to co-advised Rong Jiang who has laid the theoretical basis for population genetics using Single
Feature Polymorphisms. 10% salary is requested for Paul Marjoram and for a research associate at
50% time.
USC collaborator Magnus Nordborg: Magnus Nordborg is the authority on dealing with population
structure in Arabidopsis and has worked with the PI for several years. A recent study combined data
collected in the Natural Variation group of the PI while at Salk as a test case for association mapping
of flowering time (Hagenbald et al 2004). Magnus Nordborg will commit 10% time to this project and
be instrumental in the selection of the core set for genotyping and in the analysis of the large 384
30
RO1 GM073822 Principal Investigator/ Program Director: Borevitz, Justin
SNP panel screening. 50% salary is also requested for a research associate to work under Dr.
Nordborg.
i. RESOURCE SHARING.
Shared resources for this project include the upgraded G7 high resolution Affymetrix scanner
available at the UChicago core facility.
j. Collaborators
Jonathan Pritchard will consult on the experimental design and methods for analysis, including the
selection of a relatively unstructured sample(s) for SFP resequencing that have good power for
association mapping. Randy Scholl will help by growing all stock center lines that will be genotyped
for selection of the core set. This will enable all stocks to be distributed to the community with correct
genotype information. We will continue work with Joseph Ecker and Tom Gingeras on developments
relating to tiling arrays.
Appendix
Nordborg et al 2005
31